Dear friends,
Many AI systems have been built using data scraped from the internet. Indeed, even the cornerstone dataset for computer vision research, ImageNet, was built using images taken from the public internet. With the rise of data-centric AI, access to good data continues to grow in importance to developers.
What are the limits for scraping and using public data? Earlier this year, a United States court ruled that scraping data from websites that don’t take measures to hide it from public view doesn’t violate a law designed to thwart hackers. I believe this is a positive step for AI as well as competition on the internet, and I hope it will lead to further clarity about what is and isn’t allowed.
Many companies aim to create so-called walled gardens in which they provide exclusive access to content — even though it may be visible to all — such as social media posts or user résumés (the data at the heart of the ruling). But such data is valuable to other companies as well. For example, while LinkedIn helps users display their résumés to professional contacts, other companies might use this data to recruit potential employees, predict whether employees are likely to leave their current positions (updating a résumé is a sign), or find sales leads. Scraping the web was important in the early days of the internet to make web search viable, but as new uses come up — such as using machine learning to generate novel insights — clear rules about which data can and can’t be used, and how, become even more important.
This isn’t a simple matter. There is a fine line between protecting copyright, which incentivizes businesses to create that data, and making data widely available, which enables others to derive value from it. In addition, freely available data can be abused. For example, some face recognition companies have been especially aggressive in scraping face portraits, building systems that invade privacy.
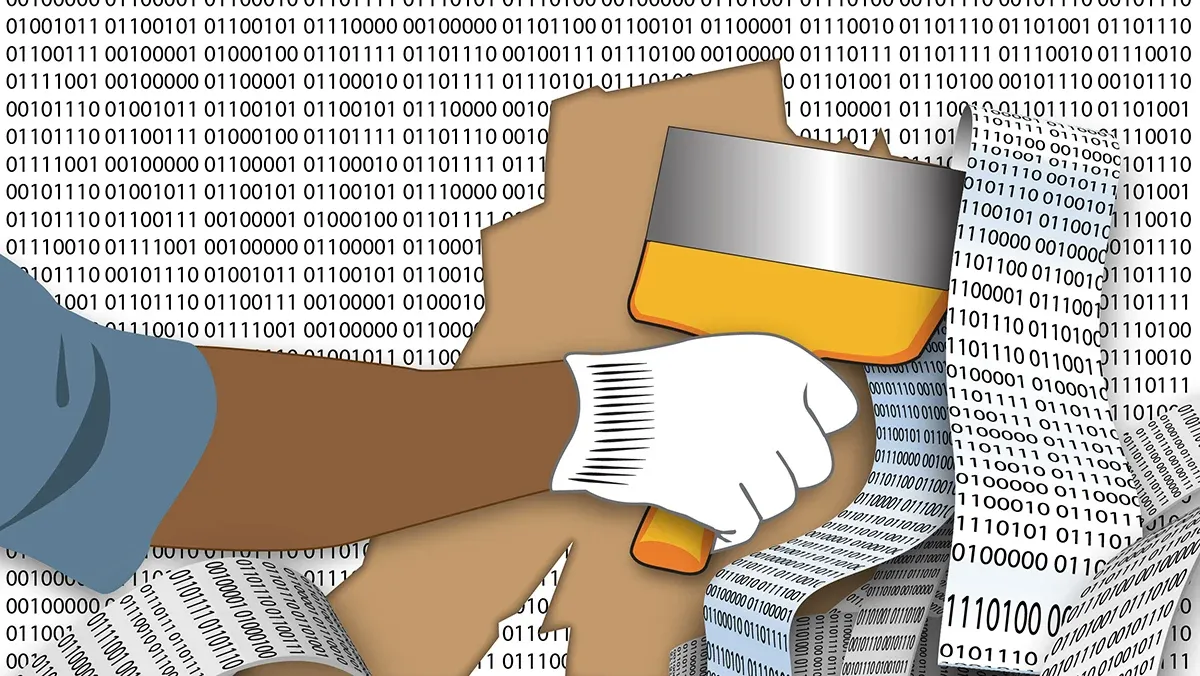
The U.S. court found that scraping data that is publicly accessible doesn’t violate the Computer Fraud and Abuse Act. This is not the same as allowing unfettered access to web scrapers. Data held behind a login wall or accessible only after agreeing to restrictive terms of service may be a different matter. (Disclaimer: Please don’t construe anything I say as legal advice.)
While this ruling may hurt companies that have built businesses on data that is fully visible to the public, overall I view it as a positive step. It will increase the free flow of information and make it easier for teams to innovate in AI and beyond. Also, knocking down part of the wall that surrounds walled gardens should increase competition on the internet. On the other hand, it increases the incentives to put data behind a login wall, where it’s no longer publicly accessible.
The issues of open versus closed data aren’t new. With the rise of mobile apps over a decade ago, web search companies worried that data would be locked within mobile apps rather than accessible on the web. This is one reason why Google invested in the Android mobile operating system as a counterweight to Apple’s iOS. Although ideas about which data should be accessible continue to shift, I continue to believe that a more open internet will benefit more people. With the rise of AI, algorithms — in addition to people — are hungry to see this data, making it even more important to ensure relatively free access.
Keep learning!
Andrew
News
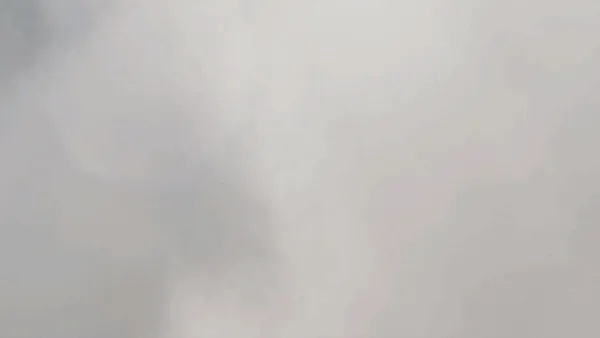
AI War Chest Grows
Western nations are making a substantial investment in AI.
What’s new: The North Atlantic Treaty Organization (NATO), which includes the United States, Canada, and much of Europe, announced a €1 billion venture capital fund that will focus on technologies including AI. The move adds to the growing momentum behind AI for warfare.
How it works: The alliance’s Innovation Fund is bankrolled primarily by 22 of the alliance’s 30 members with additional pledges from other members. It will disburse its money over 15 years.
- The fund will invest in defense-focused startups and other investment funds.
- The primary targets are AI, data processing, and autonomous machines.
- Additional targets include biotechnology, propulsion, materials, energy, and human enhancement.
Behind the news: NATO members recently boosted their individual AI budgets as well.
- In June, the UK released a defense modernization strategy centered on AI. The policy makes it easier for the military to invest in civilian AI efforts and establishes a Defence AI Centre to centralize military AI research and development.
- Also in June, Germany earmarked €500 million for research and development, including artificial intelligence. Earlier, prompted by Russia’s invasion of Ukraine, Germany had pledged 2 percent of its gross domestic product to the military — a stark reversal of the demilitarization policy it has followed since the end of World War 2.
- In 2021, the U.S. Department of Defense requested $874 million in the 2022 U.S. military budget for AI research and development.
- Looking beyond NATO, the U.S. joined Australia, India, Japan, and other Pacific nations in a pledge to work together on military AI applications by coordinating regulations on data transfers, privacy, and how AI can be used.
Why it matters: Besides autonomous weaponry, AI has numerous military applications that confer strategic and tactical advantages. In the Russian invasion of Ukraine alone, AI has been used to identify enemy soldiers, combat propaganda, and intercept communications.
We’re thinking: The rising tide of military AI adds urgency to calls for international agreements on how the technology can be used in warfare. We support the United Nations’ proposed ban on autonomous weapons.
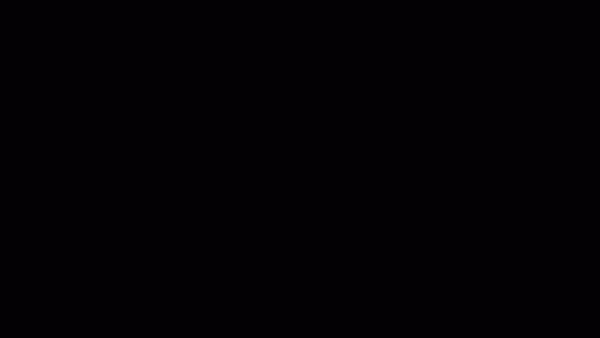
Auto Diagnosis
A drive-through system automatically inspects vehicles for dents, leaks, and low tire pressure.
What’s new: General Motors is giving its dealerships an option to install a visual inspection system from UVeye. Volvo struck a similar deal with the Tel Aviv startup in March.
How it works: UVeye’s technology is designed to cut the time it takes to inspect a vehicle from minutes, possibly hours, to seconds. The company offers three systems to be installed on a service center’s premises for an undisclosed subscription fee.
- Atlas is a large arch that identifies dents, scratches, rust, and other cosmetic damage as cars drive through. UVeye also offers a miniature version, Atlas Lite.
- Helios is a floor-mounted array of five cameras that capture an image of a vehicle’s undercarriage as it drives over. It detects damage to the vehicle’s frame, missing parts in the undercarriage, fluid leaks, and problems with braking and exhaust systems.
- Artemis uses two floor-level cameras to scan tires. It identifies the manufacturer, pressure, damage, and tread depth. It also flags mismatched tires.
Behind the news: General Motors and Volvo separately invested undisclosed sums in UVeye, as have Honda, Toyota, and Škoda, a Volkswagen subsidiary. Several General Motors dealers around the U.S. already use its technology for vehicle checkups; the new deal will make it available to all 4,000. Volvo uses UVeye scanners on its assembly lines and offers incentives to dealerships to use them as well.
Why it matters: A computer vision system that completes inspections in seconds can free mechanics to focus on more critical tasks, help dealers evaluate trade-ins, and give customers confidence that service stations are addressing real issues.
We’re thinking: Autonomous driving is the first automotive application for AI that many people think of, but other important tasks are easier to automate. Streamlining routine maintenance is one. Others include assessing insurance claims and optimizing traffic patterns.
A MESSAGE FROM DEEPLEARNING.AI
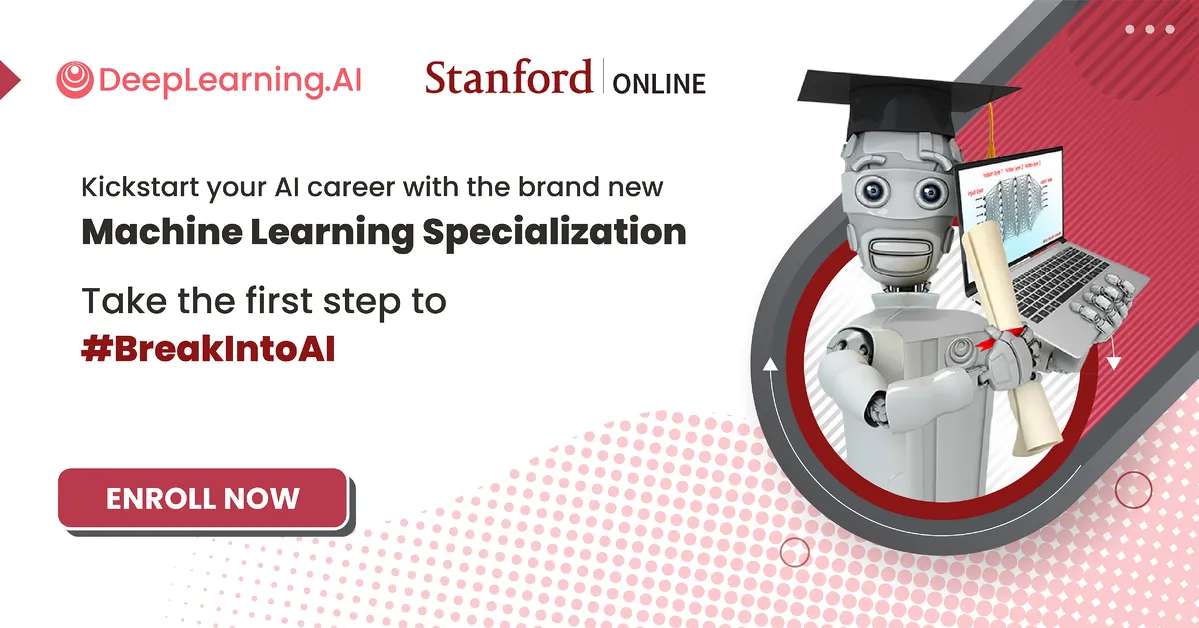
Launching today: Course 3 of the Machine Learning Specialization, “Unsupervised Learning, Recommender Systems, and Reinforcement Learning.” Learn to train models using unsupervised clustering, generate recommendations via collaborative filtering, and build deep reinforcement learning models! Enroll to #BreakIntoAI
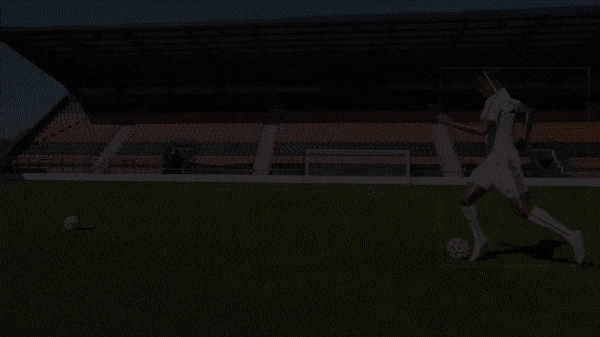
On the Ball
Neural networks are spotting up-and-coming players for some of the best teams in football (known as soccer in the United States).
What’s new: AiSCOUT uses computer vision to grade amateur footballers and recommends those who score highest to representatives of professional teams, Forbes reported.
How it works: Amateurs upload videos of themselves performing eight drills such as passing, shooting, and dribbling around cones. AiSCOUT scores the performance on a scale of 0 to 2 relative to others it has evaluated (a score of 1.7 might prompt an in-person trial with a top team).
- In addition, the system assigns up to 10 points for skills like “speed,” “dribble,” and “agility” relative to youth players who have been accepted to train with a team. Scouts and coaches can use these scores to compare prospects or track their development over time.
- A few high-profile soccer clubs have expressed enthusiasm for the app including English Premier League clubs Chelsea (which helped develop the system) and Nottingham Forest, as well as Olympiacos of the Greek Super League.
- Former English Premier League club Burnley, which also helped develop the system and used it in 2021, fell into the second tier in 2022 — a decline that raises questions about the app’s effectiveness.
Behind the news: Machine learning is being used to improve performance in a wide range of sports.
- Mustard analyzes video clips to grade baseball pitchers’ performance.
- Zone7 analyzes data from wearable sensors and athletes’ medical histories to forecast the risk that an athlete will suffer an injury in the future. It also suggests changes to an athlete's routine that may prevent injury.
- Sportlogiq analyzes broadcasts of ice hockey, soccer, and American football games to help teams, leagues, and media organizations identify promising athletes.
- SwingVision watches videos of tennis games to track shot type, speed, placement, and posture; leads and evaluates drills; and enables players to compare their performances to those of others.
Why it matters: Talent scouts have been obsessed with data since the days of pencil and paper. Machine learning can help clubs to cast a wider net and give far-flung aspirants a shot at going pro.
We’re thinking: We get a kick out of this app!
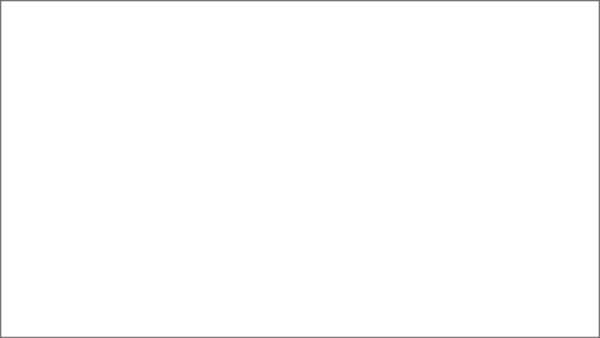
Humanized Training for Robot Arms
Robots trained via reinforcement learning usually study videos of robots performing the task at hand. A new approach used videos of humans to pre-train robotic arms.
What’s new: UC Berkeley researchers led by Tete Xiao and Ilija Radosavovic showed that real-world videos with patches missing were better than images of robot arms for training a robot to perform motor-control tasks. They call their method Masked Visual Pretraining (MVP). They also built a benchmark suite of tasks for robot arms.
Key insight: One way to train a robot arm involves two models: one that learns to produce representations of visual input and a much smaller one, the controller, that uses those representations to drive the arm. Typically, both models learn from images of a robotic arm. Surprisingly, pretraining the vision model on images of humans performing manual tasks not only results in better representations but also reduces the cost of adapting the system to new tasks. Instead of retraining the whole system on images of a new task, object, or environment, the controller alone can be fine-tuned.
How it works: The authors pretrained a visual model to reproduce images that had been partly masked by obscuring a rectangular portion at random. The pretraining set was drawn from three video datasets that include clips of humans performing manual actions such as manipulating a Rubik’s Cube. They used the resulting representations to fine-tune controllers that moved a robot arm in a simulation. They fine-tuned a separate controller for each of four tasks (opening a cabinet door as well as reaching, picking up, and relocating objects of different colors, shapes, and sizes) for each of two types of arm (one with a gripper, the other with four fingers).
- The authors pretrained the vision transformer — a masked autoencoder — to reconstruct video frames that were masked by as much as 75 percent.
- They passed representations from the transformer, along with the positions and angles of the robot arm joints, to the controllers. They used PPO to train the controllers to move the arms.
- Each controller used a different reward depending on the task. Reward functions varied depending on factors such as the distance between the robot hand or the object it was manipulating and a goal location.
Results: In all eight tasks, the authors’ approach outperformed two state-of-the-art methods that train the visual and controller models on images of robots for training. The authors compared their representations to those produced by a transformer trained on ImageNet in supervised fashion. In seven tasks, the controller that used their representations outperformed one that used the supervised transformer’s representations. In the eighth, it performed equally well. In tasks that required a four-fingered arm to pick up an object, the authors’ approach achieved a success rate of 80 percent versus 60 percent.
Yes, but: The authors didn’t compare masked pretraining on images of humans with masked pretraining on images of robots. Thus, it’s not clear whether their method outperformed the baseline due to their choice of training dataset or pretraining technique.
Why it matters: Learning from more varied data is a widely used approach to gaining skills that generalize across tasks. Masked pretraining of visual models has improved performance in video classification, image generation, and other tasks. The combination looks like a winner.
We’re thinking: Variety of data is important, but so is its relation to the task at hand. ImageNet probably is more varied than the authors’ training set of humans performing manual actions, but it’s unrelated to tasks performed by robot arms. So it stands to reason that the authors’ dataset was more effective.