Dear friends,
In the last two letters, I wrote about developing a career in AI and shared tips for gaining technical skills. This time, I’d like to discuss an important step in building a career: project work.
It goes without saying that we should only work on projects that are responsible and ethical, and that benefit people. But those limits leave a large variety to choose from. I wrote previously about how to identify and scope AI projects. This and next week’s letter have a different emphasis: picking and executing projects with an eye toward career development.
A fruitful career will include many projects, hopefully growing in scope, complexity, and impact over time. Thus, it is fine to start small. Use early projects to learn and gradually step up to bigger projects as your skills grow.
When you’re starting out, don’t expect others to hand great ideas or resources to you on a platter. Many people start by working on small projects in their spare time. With initial successes — even small ones — under your belt, your growing skills increase your ability to come up with better ideas, and it becomes easier to persuade others to help you step up to bigger projects.
What if you don’t have any project ideas? Here are a few ways to generate them:
- Join existing projects. If you find someone else with an idea, ask to join their project.
- Keep reading and talking to people. I come up with new ideas whenever I spend a lot of time reading, taking courses, or talking with domain experts. I’m confident that you will, too.
- Focus on an application area. Many researchers are trying to advance basic AI technology — say, by inventing the next generation of transformers or further scaling up language models — so, while this is an exciting direction, it is hard. But the variety of applications to which machine learning has not yet been applied is vast! I’m fortunate to have been able to apply neural networks to everything from autonomous helicopter flight to online advertising, partly because I jumped in when relatively few people were working on those applications. If your company or school cares about a particular application, explore the possibilities for machine learning. That can give you a first look at a potentially creative application — one where you can do unique work — that no one else has done yet.
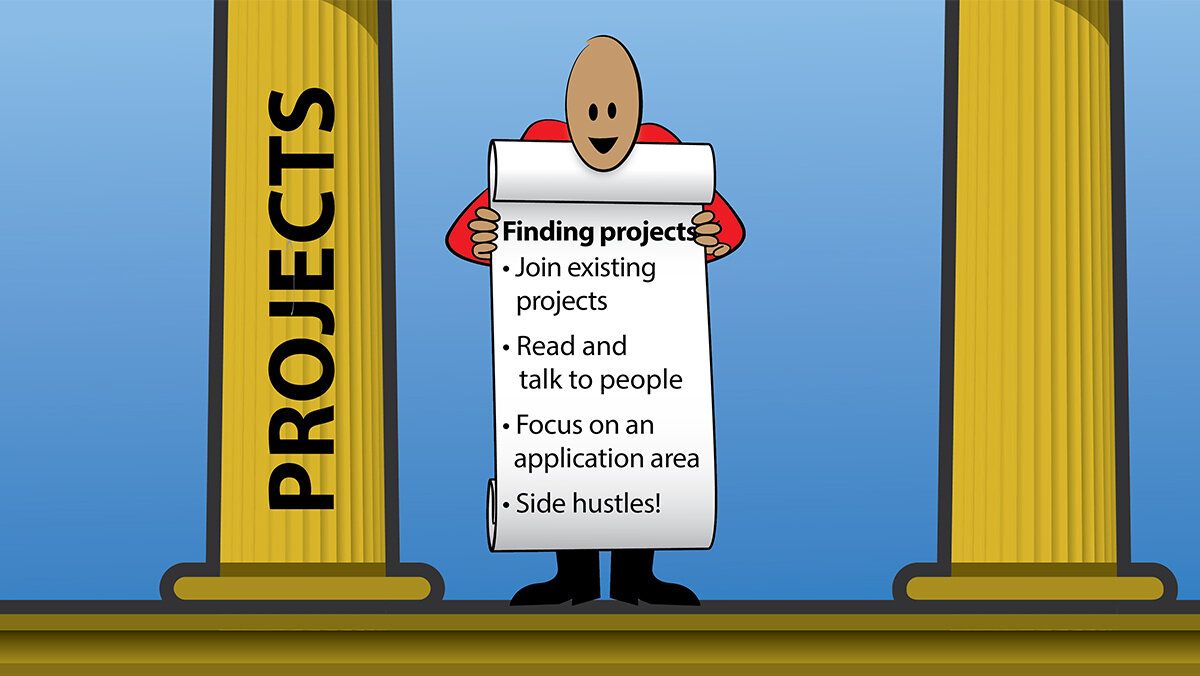
- Develop a side hustle. Even if you have a full-time job, a fun project that may or may not develop into something bigger can stir the creative juices and strengthen bonds with collaborators. When I was a full-time professor, working on online education wasn’t part of my “job” (which was doing research and teaching classes). It was a fun hobby that I often worked on out of passion for education. My early experiences recording videos at home helped me later in working on online education in a more substantive way. Silicon Valley abounds with stories of startups that started as side projects. So long as it doesn’t create a conflict with your employer, these projects can be a stepping stone to something significant.
Given a few project ideas, which one should you jump into? Here’s a quick checklist of factors to consider:
- Will the project help you grow technically? Ideally, it should be challenging enough to stretch your skills but not so hard that you have little chance of success. This will put you on a path toward mastering ever-greater technical complexity.
- Do you have good teammates to work with? If not, are there people you can discuss things with? We learn a lot from the people around us, and good collaborators will have a huge impact on your growth.
- Can it be a stepping stone? If the project is successful, will its technical complexity and/or business impact make it a meaningful stepping stone to larger projects? (If the project is bigger than those you’ve worked on before, there’s a good chance it could be such a stepping stone.)
Finally, avoid analysis paralysis. It doesn’t make sense to spend a month deciding whether to work on a project that would take a week to complete. You'll work on multiple projects over the course of your career, so you’ll have ample opportunity to refine your thinking on what’s worthwhile. Given the huge number of possible AI projects, rather than the conventional “ready, aim, fire” approach, you can accelerate your progress with “ready, fire, aim.”
Keep learning!
Andrew
News
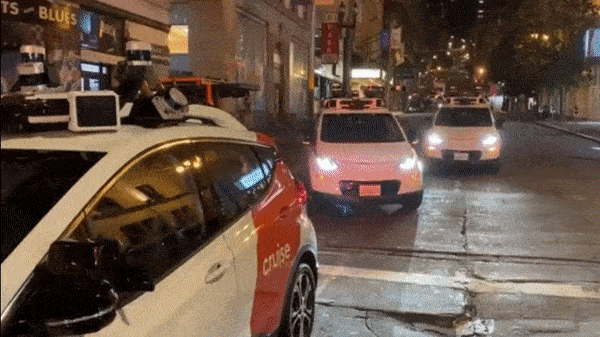
When Self-Driving Cars Won’t Drive
Dormant robotaxis are snarling traffic on the streets of San Francisco.
What’s new: Cruise self-driving cabs lately have stalled en masse, Wired reported.
What's happened: Vehicles from Cruise, a subsidiary of automotive giant General Motors, lost contact with the company’s servers at least four times since May. The outages leave the cars, which don’t carry human safety drivers, unable to move for substantial periods of time.
- On June 28, nearly 60 Cruise vehicles lost contact with company servers for 90 minutes. At least a dozen vehicles stalled in a single intersection, blocking lanes and crosswalks. The holdup blocked a street-sweeping vehicle, which is punishable by a fine. Cruise employees were unable to steer the vehicles remotely and had to drive them manually to their depot.
- On May 18, the company lost touch with the entire fleet for 20 minutes. Employees were unable to control the vehicles remotely or contact passengers.
- Similar incidents were captured by Twitter users on June 24 and June 21.
Behind the news: On June 2, Cruise acquired the first-ever permit to collect robotaxi fares in San Francisco. The permit allows 30 vehicles to operate between 10 p.m. and 6 a.m. They’re authorized to drive up to 30 miles per hour in clear weather.
Why it matters: Rolling out self-driving cars has proven to be more difficult than many technologists realized. Cruise has made great progress with its taxi program, reducing the hazard of autonomous vehicles in motion sufficiently to gain a permit to operate on public roads. But centralized control brings its own hazards — and a fat target for hackers and saboteurs.
We’re thinking: Why do self-driving cars need internet access to drive? Many autonomous systems actually rely on remote humans to monitor and help them operate safely. A failsafe for loss of contact with remote servers is in order, but this is very challenging with today’s technology.
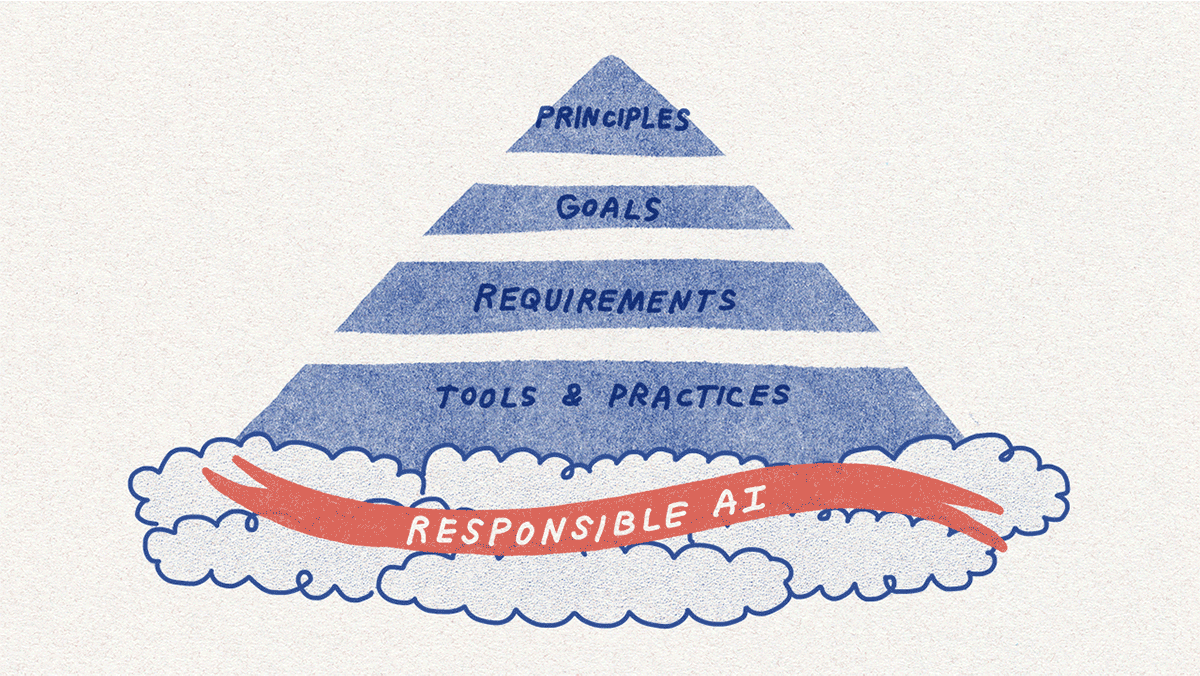
Ethical AI 2.0
Microsoft tightened the reins on both AI developers and customers.
What’s new: The tech titan revised its Responsible AI Standard and restricted access to some AI capabilities accordingly.
Taking responsibility: The update is intended to support six core values.
- Accountability: Developers should assess how a system will affect society, whether it’s a valid solution to the associated problem, and who bears responsibility for the system and its data. Additional scrutiny should be devoted to AI products in socially sensitive areas like finance, education, employment, healthcare, housing, insurance, or social welfare.
- Transparency: Systems should be thoroughly documented. Users should be informed that they are interacting with AI.
- Fairness: Developers should assess a system’s fairness to different demographic groups and actively work to minimize differences. Developers should publish details to warn users of any risks they identify.
- Reliability and Safety: Developers should determine a system’s safe operating range and work to minimize predictable failures. They should also establish procedures for ongoing monitoring and guidelines for withdrawing the system should unforeseen flaws arise.
- Privacy and Security: Systems should comply with the company’s privacy and security policies, ensuring that users are informed when the company collects data from them and that the resulting corpus is protected.
- Inclusiveness: Systems should comply with inclusiveness standards such as accessibility for people with disabilities.
Face off: To comply with its new guidelines, the company limited AI services offered via its Azure Cloud platform.
- New customers of the company’s face recognition and text-to-speech services must apply for access.
- The face recognition service no longer provides estimates of age, gender, or emotion based on face portraits. Existing customers will be able to use these capabilities until June 2023.
Behind the news: Microsoft published its first Responsible AI Standard in 2019 but concluded that the initial draft was vague. The new version is intended to give developers clearer directions for compliance. To that end, the company also provides nearly 20 tools intended to aid developers in building responsible AI systems. For instance, HAX Workbook helps make AI systems easier to use, InterpretML helps explain model behavior, and Counterfit stress-tests security.
Why it matters: Regulation in the United States and elsewhere lags rising concern that AI is growing more capable of causing harm even as it becomes enmeshed in everyday life. Microsoft’s latest moves represent a proactive effort to address the issue.
We’re thinking: Hundreds of guidelines have been drafted to govern AI development. The efforts are laudable, but the results are seldom actionable. We applaud Microsoft for working to make its guidelines more concrete, and we’re eager to see how its new standards play out in practice.
A MESSAGE FROM DEEPLEARNING.AI
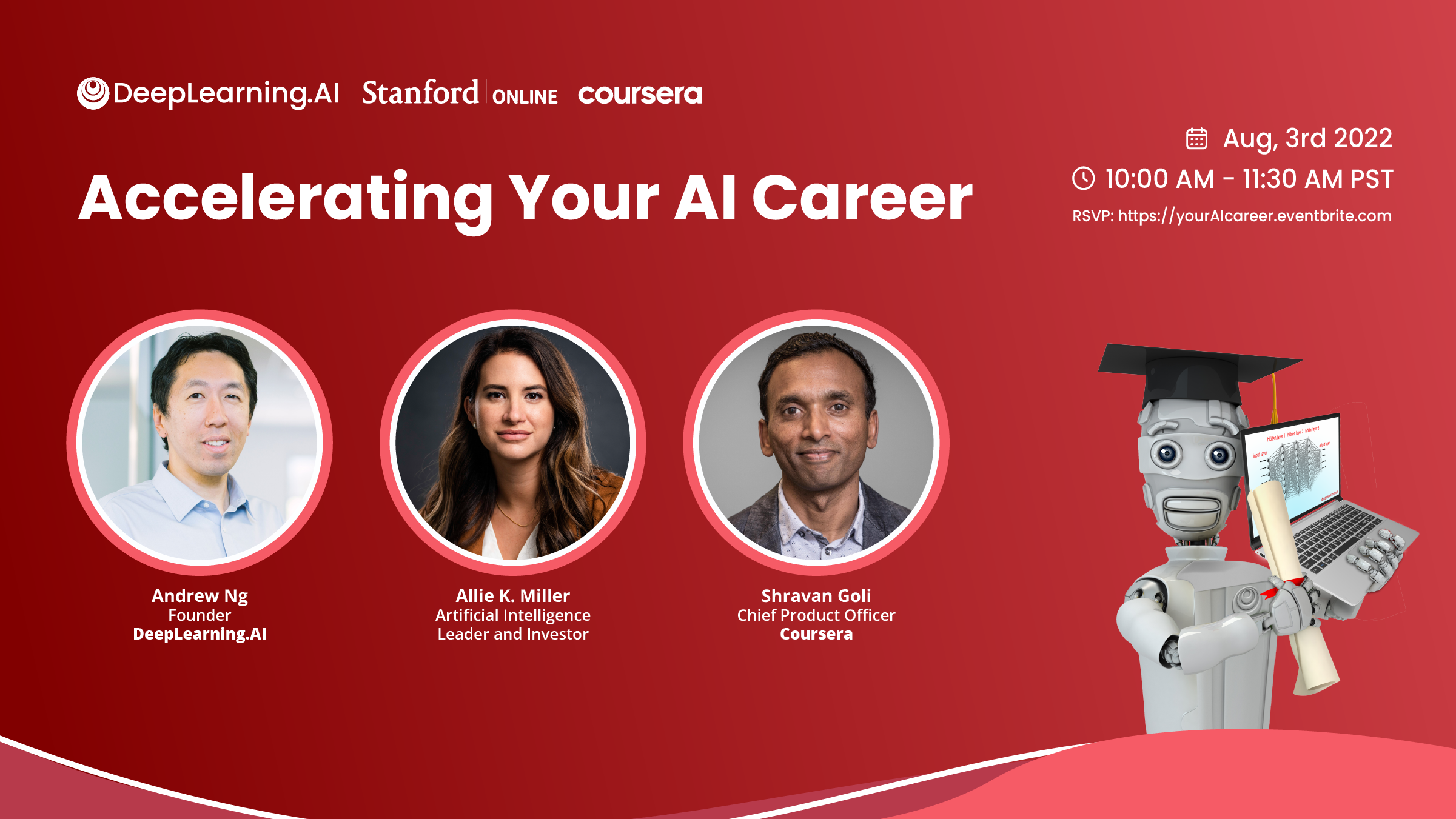
Join us on August 3, 2022, for Accelerating Your AI Career! Andrew Ng and other industry experts will discuss core skills for a career in machine learning and how learners can develop them. A demo of the Machine Learning Specialization will follow.
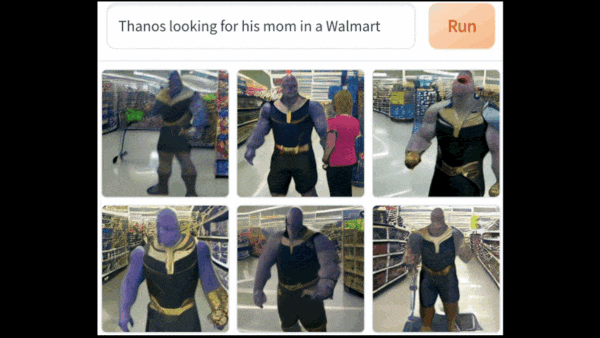
Text-to-Image Goes Viral
A homebrew re-creation of OpenAI’s DALL·E model is the latest internet sensation.
What’s new: Craiyon has been generating around 50,000 user-prompted images daily, thanks to its ability to produce visual mashups like Darth Vader ice fishing and photorealistic Pokemon characters, Wired reported. You can try it here.
How it works: U.S. machine learning consultant Boris Dayma built Craiyon, formerly known as DALL·E Mini, from scratch last summer. It went viral in early June following upgrades that improved its output quality.
- Dayma fine-tuned a pretrained a VQGAN encoder/decoder to reproduce input images and to generate images that fooled a separate discriminator (a convolutional neural network) into classifying them as real images.
- He trained a BART, given a caption, to generate a sequence of tokens that matched VQGAN’s representation of the corresponding image. The training set comprised 30 million captioned images from public datasets that were filtered to remove sexual and violent imagery.
- At inference, given input text, BART’s encoder produces a sequence of tokens. Given that sequence, its decoder predicts a probability distribution for each successive image token. It uses those distributions to generate several representations for possible images.
- Given the representations, the VQGAN decoder generates images. CLIP ranks them on how closely they match the text, and outputs the top nine.
Behind the news: Fans of the word-guessing game Wordle may enjoy Wordalle, which shows players six images generated by Craiyon and asks them to guess the prompt.
Why it matters: Advances in machine learning are unlocking new ways for people to amuse themselves, from generating images of imaginary pizzas to making superheroes lip-synch popular songs. Enabling the internet audience to remix popular culture in unprecedented ways unleashes imagination and good humor worldwide.
We’re thinking: OpenAI says it controls access to DALL·E out of concern that people might use it to indulge their worst impulses. Craiyon’s deluge of delightful output is an encouraging counterpoint.
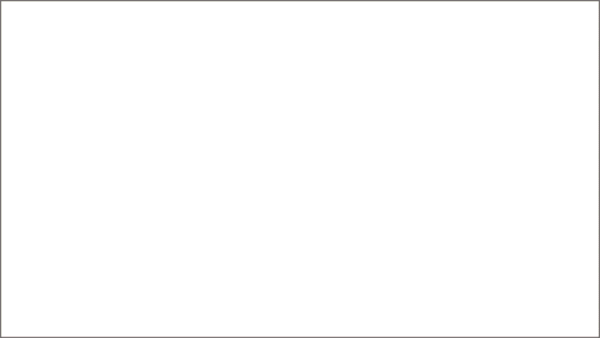
Tradeoffs for Higher Accuracy
Vision models can be improved by training them on several altered versions of the same image and also by encouraging their weights to be close to zero. Recent research showed that both can have adverse effects that may be difficult to detect.
What’s new: Randall Balestriero, Leon Bottou, and Yann LeCun at Meta found that using augmented data and the form of regularization known as weight decay, though they typically boost performance overall, can degrade a model’s performance on some classes.
Key insight: Augmenting training images by cropping, coloring, and otherwise altering them varies patterns in their pixels, helping models learn to generalize beyond the specific examples in the dataset. For instance, if a model uses stripes to classify zebras, then randomly altering color values in training images of zebras can help it learn to recognize zebras despite color variations in input images at inference. However, altering colors in training images may also disrupt the model’s ability to learn from certain patterns. If a model uses color to classify basketballs, then changing the colors in training images of basketballs may render it unable to distinguish basketballs from other spherical objects. Weight decay, which helps models generalize by encouraging weights to be closer to zero during training, may raise similar issues. Both weight decay and pruning reduce the impact of the lowest weights. Previous work showed that pruning, which zeroes out weights that are near zero after training, adversely affects some classes more than others. Shifting low weights closer to zero may do the same.
How it works: The authors trained separate sets of roughly 20 ResNets on ImageNet images that had been altered by randomly cropping, blacking out a rectangle, and adjusting color by changing brightness, contrast, saturation, and hue. They tested the models on ImageNet.
- The authors trained different sets of models on varying amounts of each alteration; for instance, cropping images by different percentages. They averaged each set’s accuracy on each class and graphed the results.
- They ran similar experiments using weight decay instead of data augmentation: They trained different sets of models on varying amounts of weight decay and averaged their accuracy on each class.
Results: Data augmentations increased the models’ average accuracy on some classes and decreased it on others. For instance, models trained on a dataset from which four-fifths of each image had been cropped achieved 56 percent average accuracy on pickup trucks and 59 percent on academic gowns. Cropping the dataset by three-fifths boosted average accuracy to 75 percent on trucks but cut it to 46 percent on gowns. Weight decay also affected some classes more than others. For example, with very little weight decay, average accuracy was nearly the same (around 47 percent) on gongs and miniature poodles. But with a high weight decay factor, average accuracy reached 72 percent on gongs but plummeted to 22 percent on poodles.
Why it matters: This work raises caution around techniques that improve overall performance. Although a model’s performance is very high on average, its performance on a given class may be much lower.
We’re thinking: In last year’s Data Centric AI Competition, a top-ranked team team augmented data differently depending on its class. For instance, flipping Roman numeral I horizontally doesn’t affect the label, but flipping Roman numeral IV horizontally changes the label from 4 to 6. The team determined appropriate augmentations manually rather than using one-size-fits-all alterations. This work adds credence to the value of such approaches.