Dear friends,
With the pandemic easing in the United States and Canada, I’ve been traveling more in the last two weeks. I spoke at TED 2022 in Vancouver and ScaleUp:AI in New York and attended a manufacturing conference in California.
What a pleasure it was to see people in 3D! In the days before Covid, serendipitous conversations were a large part of how I kept up with what’s happening in the world. I’ve really missed these meetings.
It was great to hear former world chess champion and Russian dissident Garry Kasparov speak and to chat with him afterward about Russia’s invasion of Ukraine. (I largely agree with his views.) I enjoyed conversing with astronaut Chris Hadfield about property rights on the moon, MIT professor Ariel Ekblaw about living in space, and neuroscientist Frances Chance about when we might develop a theory of how the mind works. I saw AI artist Sophia Crespo present her generated creatures and heard venture capitalists George Mathew and Lonne Jaffe talk about investing in AI startups.
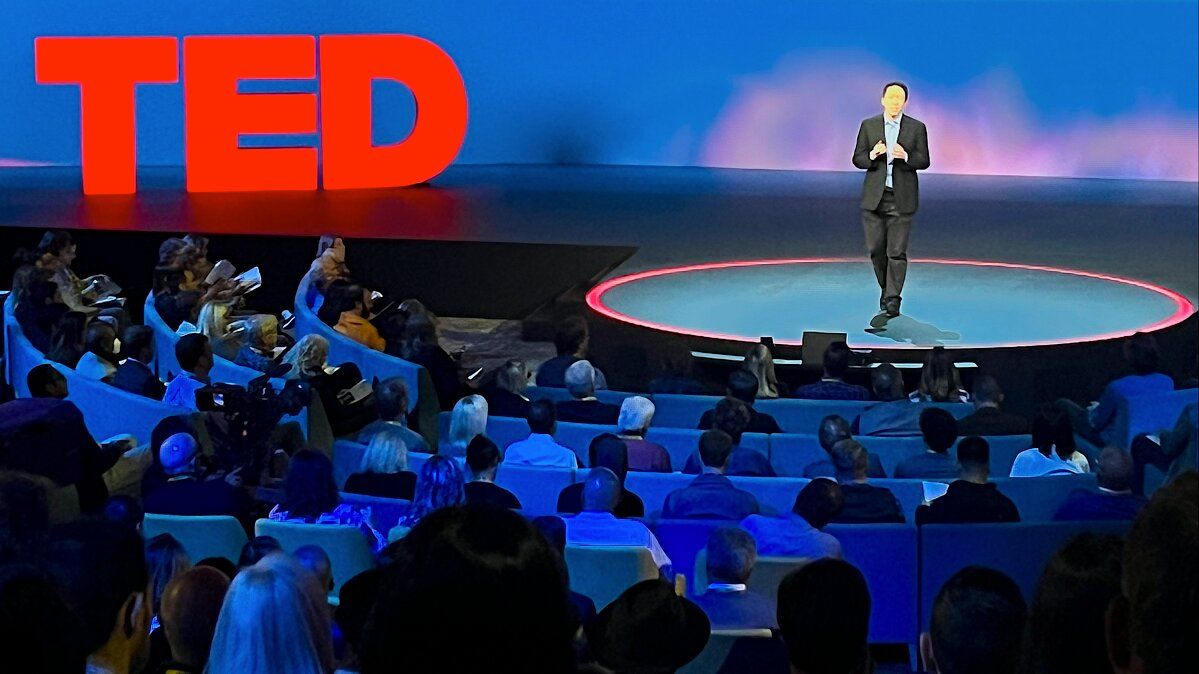
I found these conversations tremendously stimulating, and I came away thinking about some observations with respect to AI.
- To the general public, AI is still mysterious and inaccessible. Many people think that AI means AGI (artificial general intelligence), which remains far away. They don’t understand how deeply AI is already embedded in society. People would be better off if they made personal and business decisions — Should I study radiology? Should I cultivate my company’s ability to produce data? — based on realistic expectations for the future. So let’s get out there and keep helping people to shape a realistic perspective.
- Much of the infrastructure for building and deploying AI systems, such as MLOps tools, remains to be built. Despite the valiant efforts of many startups and cloud companies, it will be many years before the ecosystem of software infrastructure settles. Infrastructure for data manipulation and storage, and for data-centric approaches in particular, will play a large role.
- The community of artists who are using AI to create images or music is small but growing quickly. Some are getting by selling NFTs of their work. I’m pleased that artists can make money this way, though I’m nervous about how scalable this revenue stream will be. I hope that individuals with means will continue to support the arts regardless of the resale value of NFTs.
- Many people in the space industry are excited to take advantage of AI. There are myriad unsolved problems in, say, getting humans to Mars and back, from generating thrust to ensuring a soft landing. These are great opportunities for the AI community.
Going to these in-person events has me looking forward to a time, hopefully soon, when DeepLearning.AI and our ambassadors can hold more in-person events safely. I realize that the pandemic still varies widely in different regions. I hope you’ll enjoy reconnecting in person when it’s safe for you to do so, and benefit from the joyful conversations that contribute so much to learning.
Keep learning!
Andrew
News
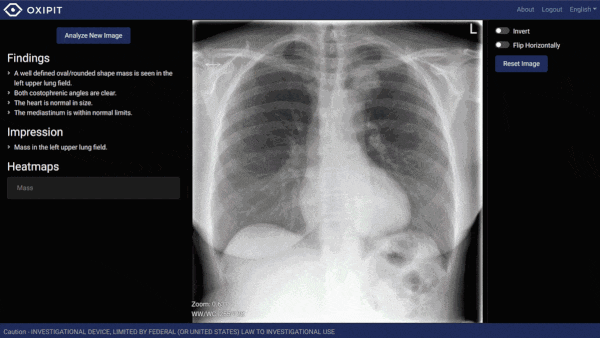
AI Enters the Radiology Department
The European Union approved for clinical use an AI system that recognizes normal chest X-rays.
What’s new: ChestLink is the first autonomous computer vision system to earn the European Economic Area’s CE mark for medical devices, which certifies that products meet government requirements for health and safety. The mark enables Oxipit, the Lithuanian startup that makes the system, to deploy it in the 27 E.U. countries plus Iceland, Liechtenstein, Norway, Switzerland, and Turkey.
How it works: ChestLink uses a previous Oxipit product, ChestEye, to scan for 75 abnormalities such as edema and tuberculosis. If it finds none, it generates a medical report. Otherwise it forwards the image to a radiologist for review.
- Prior to deployment in a given clinic, the company runs X-rays produced in that setting through the system to find the percentage of abnormality-free images it can recognize with high certainty. After deployment, Oxipit evaluates the system’s efficacy before letting it run autonomously.
- Oxipit tested ChestLink for a year at several clinics using 500,000 medical images.
- The company aims to deploy it autonomously next year, after which it hopes to gain approval by the United States Food and Drug Administration.
Why it matters: Reading X-ray images is highly subjective. Moreover, a radiologist’s judgment can vary as fatigue sets in over the course of a working day. By identifying and reporting on normal images, this system could help radiologists focus on the cases that need the most attention.
We’re thinking: Even the best AI systems for diagnosing chest X-rays fall short of a board-certified radiologist’s accuracy. Training AI to recognize problem-free images, which are less ambiguous, is a clever approach.
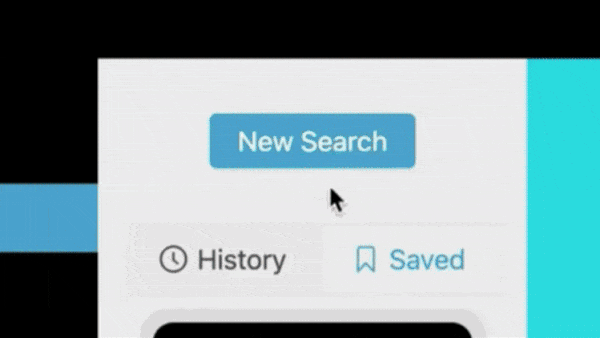
Seeing Through the Fog of War
Face recognition is identifying people who have been killed, displaced, or recorded perpetrating alleged war crimes in Russia’s invasion of Ukraine.
What’s new: Clearview AI, a startup that has been criticized for harvesting online images without subjects’ permission, made its face recognition system freely available to the Ukrainian government, The New York Times reported. Researchers unaffiliated with the Ukrainian government are using similar tools to analyze images of the conflict.
How it works: Clearview has created 200 accounts at five Ukrainian agencies. Officials have used its app to conduct over 5,000 searches.
- In emails provided to the Times, Ukrainian national police described using the app to identify a dead Russian soldier by matching the fighter’s image to pictures uploaded to Odnoklassniki, a Russian social media site. They also identified prisoners of war and confirmed the identities of noncombatants traveling within Ukraine.
- A researcher with the Dutch investigative group Bellingcat used FaceClone, a Russian face recognition app trained on data from the social media site VKontakte, to identify Russian soldiers in videos that showed them mailing items looted from Ukrainian homes.
- Earlier in the conflict, Bellingcat and Tactical Systems, a military training company, used Microsoft’s face recognition tech to debunk claims that a Russian pilot captured in Ukraine had been photographed alongside Vladimir Putin in 2017.
- Analysts believe that Russian forces and sympathizers are using the technology in similar ways. “I’m sure there are Russian analysts tracking Twitter and TikTok with access to similar if not more powerful technology, who are not sharing what or who they find so openly,” Ryan Fedasiuk, a military research analyst, told Wired.
Yes, but: Face recognition can produce erroneous output. Amid military conflict, such errors — combined with wartime pressures — may cause people to be misidentified as war criminals, spies, or deceased.
Behind the news: AI is being used to analyze a variety of data types flowing out of Ukraine.
- PrimerAI retrained a natural language model to recognize Russian slang and military jargon so it can transcribe unencrypted radio transmissions that have been intercepted and posted online.
- Researchers at the UC Berkeley trained computer vision models on images from synthetic-aperture radar mounted on satellites, which can see through clouds, to identify damaged buildings.
Why it matters: The invasion of Ukraine — captured in an avalanche of photos, videos, aerial imagery, and radio transmissions shared on social media — is one of the most data-rich conflicts in history. Given this grim corpus, face recognition and other AI techniques can help to sketch a more complete picture of the battlefield.
We’re thinking: The ability to unmask war criminals and thereby help bring them to justice offers solace amid unspeakable misery. We hope it also will deter other offenders. To recover from this tragedy will require still greater ingenuity and fortitude. We join the international community in calling on Vladimir Putin to withdraw Russian forces immediately.
A MESSAGE FROM DEEPLEARNING.AI
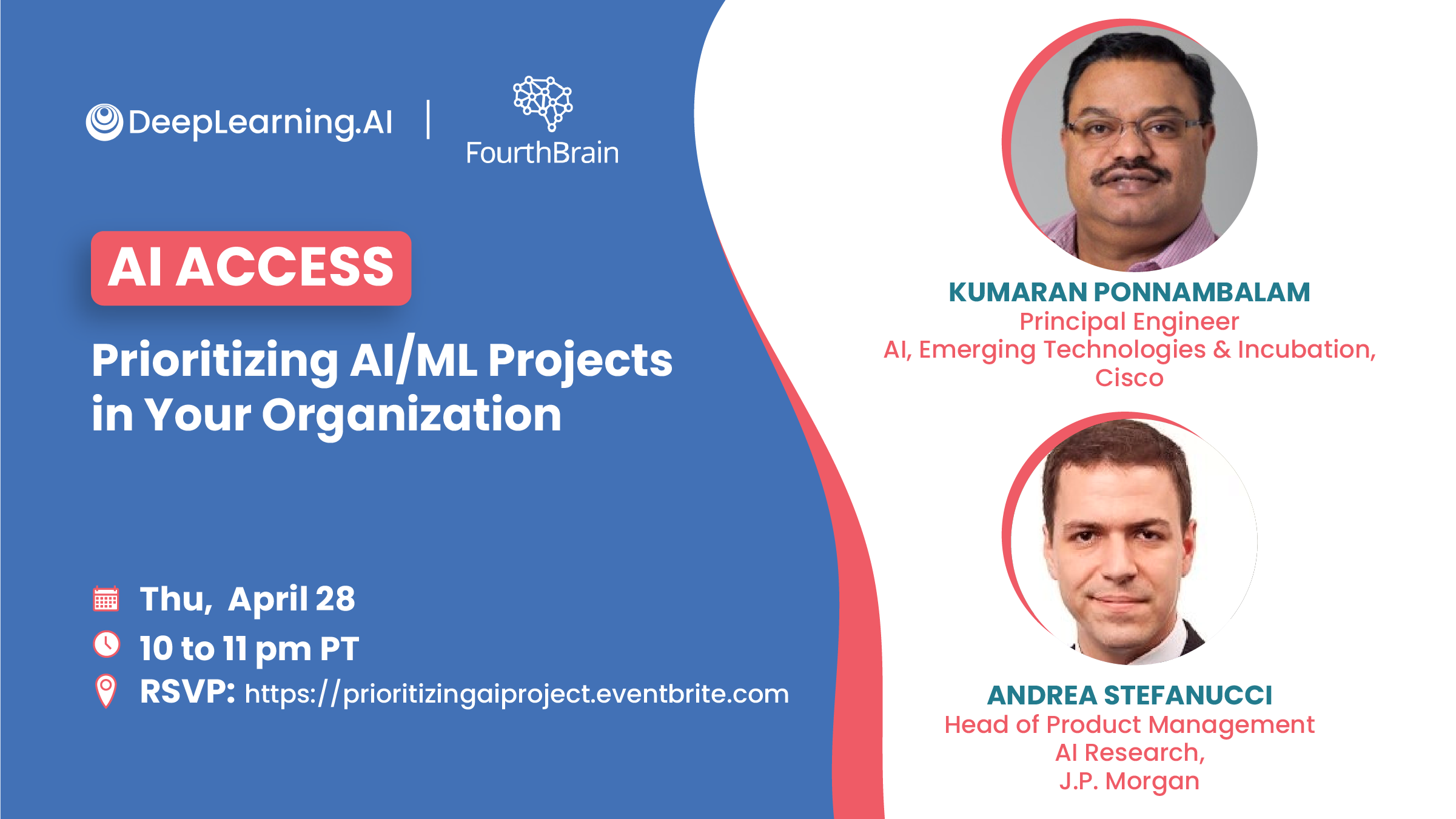
Many organizations embark on machine learning projects only to encounter roadblocks and eventually fail. Join us for a live event on how to maximize your potential for success.
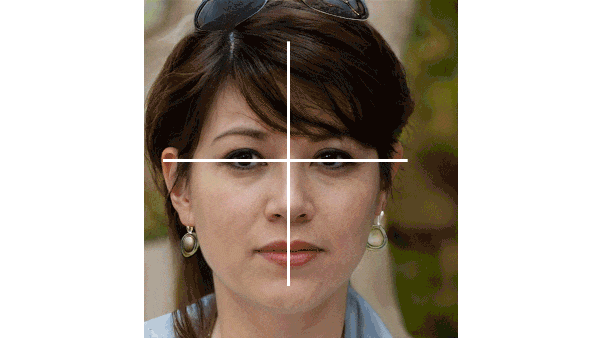
Your Salesbot Connection
Marketers are using fake social media personas — enhanced by AI-generated portraits — to expand their reach without busting their budgets.
What’s new: Renee DiResta and Josh Goldstein at Stanford Internet Observatory combed LinkedIn and discovered over 1,000 fake profiles with false faces they believe to have been produced using generative adversarial networks, the radio network NPR reported.
How it works: Companies hire independent marketers to expand their markets by messaging potential customers on social media. These marketers use fake profiles to send sales pitches. Responses are routed to a salesperson at the original company.
- LIA (for LinkedIn Lead Generation Assistant) sells access to online avatars that “love nothing more than prowling LinkedIn profiles to find high-quality, engaged leads” for $300 a month.
- Renova Digital enables its customers to control two automated avatars for $1,300 a month. It doesn’t use deepfakes as profile pictures.
- Alerted by DiResta and Goldstein, LinkedIn deleted profiles that violated its community standards. It removed 15 million fake profiles during the first six months of 2021, nearly all of which were blocked automatically at registration or following suspicious behavior.
Spot the fake: DiResta and Goldstein shared tips for recognizing forged LinkedIn profiles.
- Portraits produced by generative adversarial networks show telltale signs like eyes that align horizontally with the image’s center, ears adorned with asymmetrical jewelry, and wayward strands of hair.
- Fake profiles often list employers — commonly major companies like Amazon and Salesforce — but little detail about the roles.
- Fake educational histories may contain inaccuracies. For instance, several profiles discovered by the researchers mentioned a bachelor’s degree in business administration from a school that didn’t offer such a degree.
Why it matters: In the era of social media, companies have access to far more potential customers than their sales teams could possibly reach. This gives them ample incentive to look to AI for assistance. However, the risk of blowback for deceiving the public may outweigh the prospective gains.
We’re thinking: Need we say it? Deceptive sales tactics are unacceptable no matter how cool your technology may be.
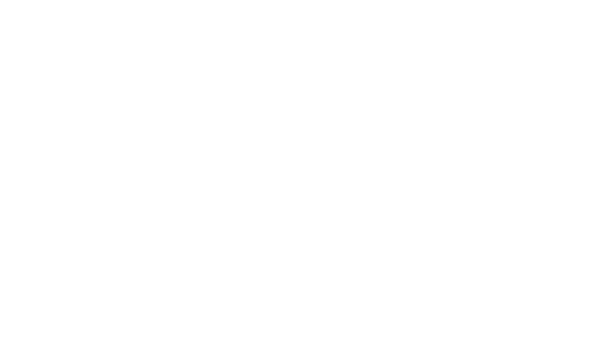
From Sequences to Symbols
Given a sequence of numbers, neural networks have proven adept at discovering a mathematical expression that generates it. New work uses transformers to extend that success to a further class of expressions.
What’s new: A team at Meta (formerly Facebook) led by Stéphane d’Ascoli and Pierre-Alexandre Kamienny introduced Deep Symbolic Regression, training models to translate integer and float sequences to mathematical expressions. Unlike earlier work, their approach is able to find functions in which terms in a sequence depend on previous terms (such as the Fibonacci sequence un = un-1 + un-2). You can try out an interactive demo here.
Key insight: Transformers excel at learning underlying patterns in natural language. Converting a sequence of numbers into a mathematical expression is analogous to translating one natural language into another.
How it works: Given a sequence of numbers, a transformer learned to generate a function made up of operators (such as add, multiply, modulo, and square root), constants, the index of the term to be computed, and references to previous terms.
- To train the model, the authors generated 5 million expressions by sampling from possible values (operators, constants, and so on), assembling them in the proper format, and sampling any values required to start the sequence. Then they computed each expression’s results, generating sequences of random length between 5 and 30 terms.
- During training, the loss function encouraged the generated function to match the true function.
- The authors evaluated their approach according to the next 10 terms in a given sequence. This method was preferable to comparing generated expressions to their true equivalents, as a given expression can take various equivalent forms (by, say, swapping the order of two terms in a sum).
Results: The authors compared their symbolic approach with a numeric model (a transformer trained to predict the next 10 terms in a sequence). Generating expressions of up to 10 operators that resulted in integer sequences, the symbolic model achieved 78.4 percent accuracy compared to the numeric model’s 70.3 percent. Generating expressions that resulted in float sequences — a more difficult task — the symbolic model achieved 43.3 percent accuracy compared to the numeric model’s 29 percent. The symbolic model also outperformed Mathematica’s built-in methods for deriving functions from sequences, tested on sequences sampled from the Online Encyclopedia of Integer Sequences (OEIS). Generating 10 terms that followed sequences of length 15, the numeric and symbolic models achieved accuracies of 27.4 percent and 19.2 percent respectively. Mathematica’s FindSequenceFunction and FindLinearRecurrence achieved 12 percent and 14.8 percent.
Yes, but: To rule out arbitrary sequences such as the digits of pi, the authors selected OEIS sequences classified as easy; that is, results of expressions deemed easy to compute and understand. Finding expressions that yield more complicated sequences might strain the authors’ approach.
Why it matters: Machine learning research struggles with abstract reasoning tasks. Mathematical symbols may be a piece of the solution.
We’re thinking: 2, 4, 6, 8, who do we appreciate? Transformers!