Dear friends,
I’ve always thought that building artificial general intelligence — a system that can learn to perform any mental task that a typical human can — is one of the grandest challenges of our time. In fact, nearly 17 years ago, I co-organized a NeurIPS workshop on building human-level AI.
Artificial general intelligence (AGI) was a controversial topic back then and remains so today. But recent progress in self-supervised learning, which learns from unlabeled data, makes me nostalgic for the time when a larger percentage of deep learning researchers — even though it was a very small group — focused on algorithms that might play a role in mimicking how the human brain learns.
Obviously, AGI would have extraordinary value. At the same time, it’s a highly technical topic, which makes it challenging for laypeople — and even experts — to judge which approaches are feasible and worthwhile. Over the years, the combination of AGI’s immense potential value and technical complexity has tempted entrepreneurs to start businesses on the argument that, if they have even a 1 percent chance of success, they could be very valuable. Around a decade ago, this led to a huge amount of hype around AGI, generated sometimes by entrepreneurs promoting their companies and sometimes by business titans who bought into the hype.
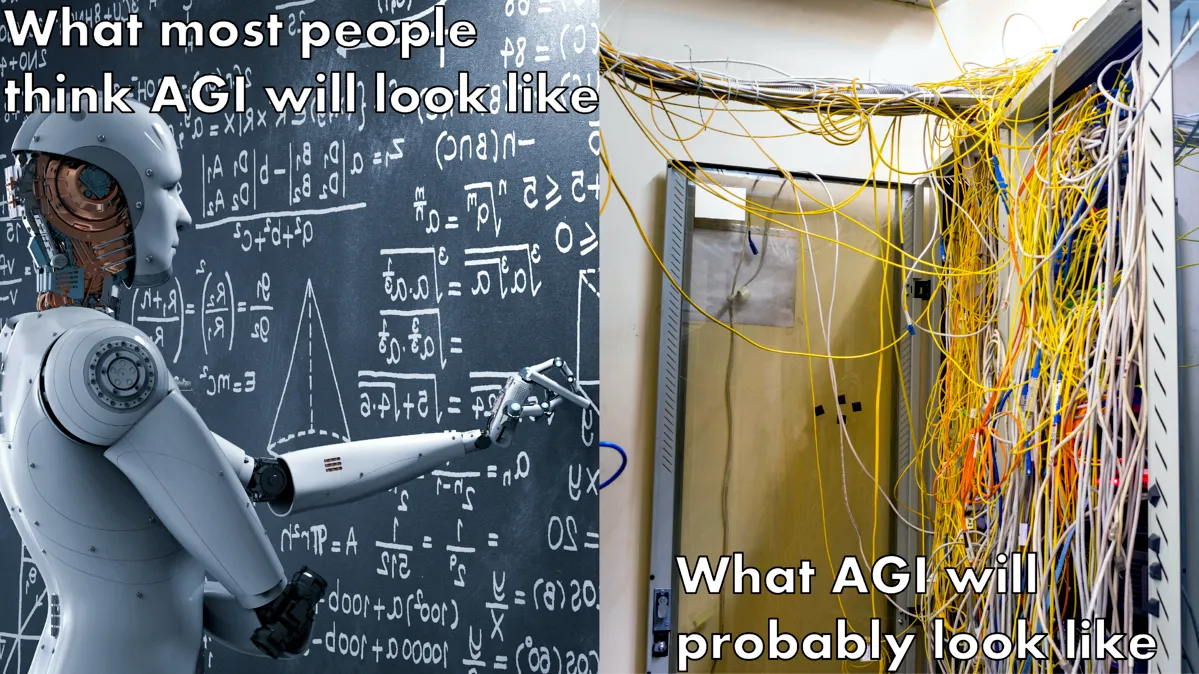
Of course, AGI doesn’t exist yet and there’s no telling if or when it will. The volume of hype around it has made many respectable scientists shy away from talking about it. I’ve seen this in other disciplines as well. For decades, overoptimistic hopes that cold fusion would soon generate cheap, unlimited, safe electricity have been dashed repeatedly so that, for a time, even responsible scientists risked their reputations by talking about it.
The hype around AGI has died down compared to a few years ago. That makes me glad, because it creates a better environment for doing the work required to make progress toward it. I continue to believe that some combination of learning algorithms, likely yet to be invented, will get us there someday. Sometimes I wonder whether scaling up certain existing unsupervised learning algorithms would allow neural networks to learn more complex patterns, for instance self-taught learning and self-supervised learning. Or — to go farther out on a limb — sparse coding algorithms that learn sparse feature representations. I look forward also to a foundation model that can learn rich representations of the world from hundreds of thousands of hours of video.
If you dream of making progress toward AGI yourself, I encourage you to keep dreaming! Maybe some readers of The Batch one day will make significant contributions toward this grand challenge.
Keep learning!
Andrew
News
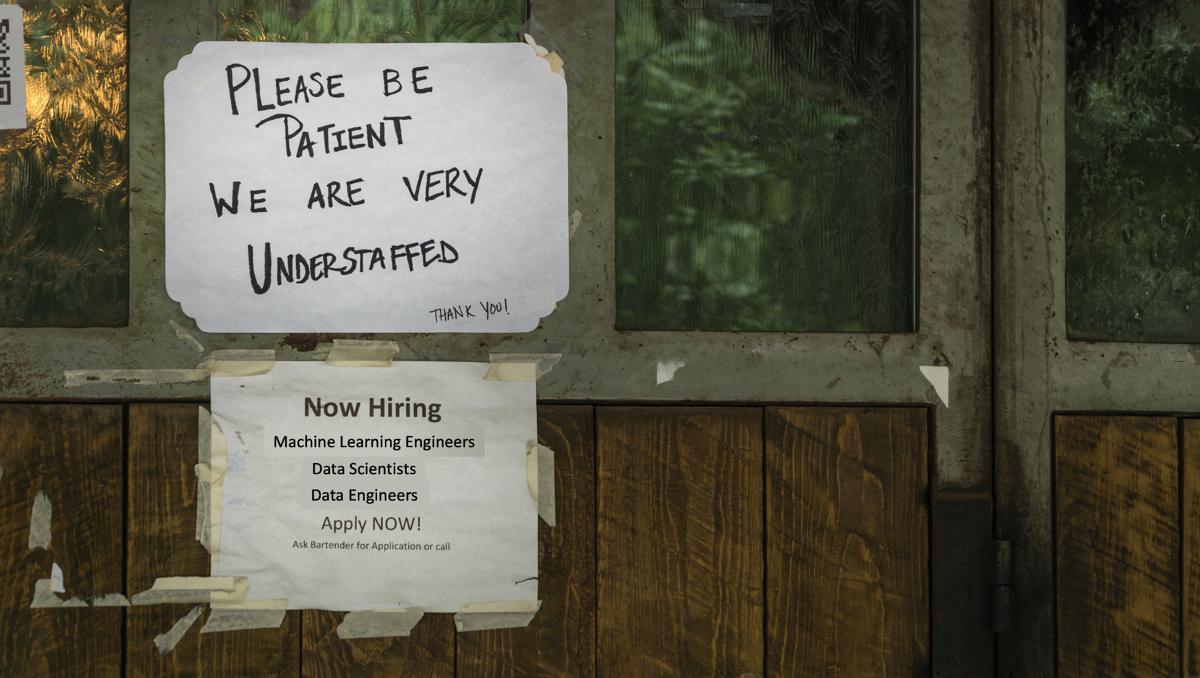
Help Wanted: AI Developers
A shortfall in qualified AI professionals may be a windfall for aspiring engineers.
What’s new: Hiring managers are struggling to find machine learning engineers amid an ongoing, global talent shortage, Business Insider reported. Some employers are going the extra mile to distinguish themselves from competitors in the eyes of potential employees.
Supply and demand: The number of new graduates with machine learning backgrounds is not keeping pace with demand for their skills.
- “Five or so years ago, many companies were just scratching the surface of AI capabilities,” said Narek Verdian, chief technology officer at Barcelona-based Glovo, which makes a shopping app. “Now AI is ingrained in every industry and transforming the way we do things every day.”
- The Covid-19 pandemic disrupted the job market as many firms stopped hiring. Now they’re playing catch-up, said Kristianna Chung, head of data science at Harnham, a New York recruitment firm.
- A wider range of applications than ever before can take advantage of machine learning, said Catherine Breslin, founder of the UK consultancy Kingfisher Labs. That’s stretching the pool of potential hires even thinner.
- Candidates qualified for junior positions are as hard to find as those for more experienced roles, observed Angie Ma, co-founder of London software and consulting startup Faculty AI.
Fringe benefits: High demand for machine learning engineers is empowering qualified applicants to secure perks.
- Machine learning engineers increasingly demand to work remotely as they take up residence outside of traditional tech centers. Yet salaries are still guided by the high cost of living in Silicon Valley, according to Breslin.
- Candidates are asking for company details such as funding sources and growth plans from the beginning of the hiring process, Chung said.
- Firms hoping to attract candidates and improve retention should allow their employees to publish research and take time off to pursue side projects, advised Joshua Saxe, chief scientist at UK software firm Sophos.
Behind the news: Recent studies confirm both the rising demand for machine learning engineers and the scarcity of qualified candidates.
- A 2021 LinkedIn study found that machine learning engineer was the fourth fastest-growing job title in the U.S. between January 2017 and July 2021.
- Shortage of talent is causing companies in a variety of industries to fall short of their automation goals, a 2020 Deloitte survey determined.
- A 2020 report concluded that the scarcity of machine learning talent was behind an exodus of AI-focused professors from academia to industry between 2004 and 2018.
Why it matters: The hiring boom in machine learning and data science isn’t new, but it shows no sign of slowing and may be intensifying as the pandemic wanes. It’s a great time for candidates to approach employers and for academic institutions to meet rising demand with strong educational programs.
We’re thinking: The labor shortage is great for employees in the short term, but it also holds back AI development from reaching its full potential. It’s high time for everyone to build AI capacity, from individuals to businesses to institutions.
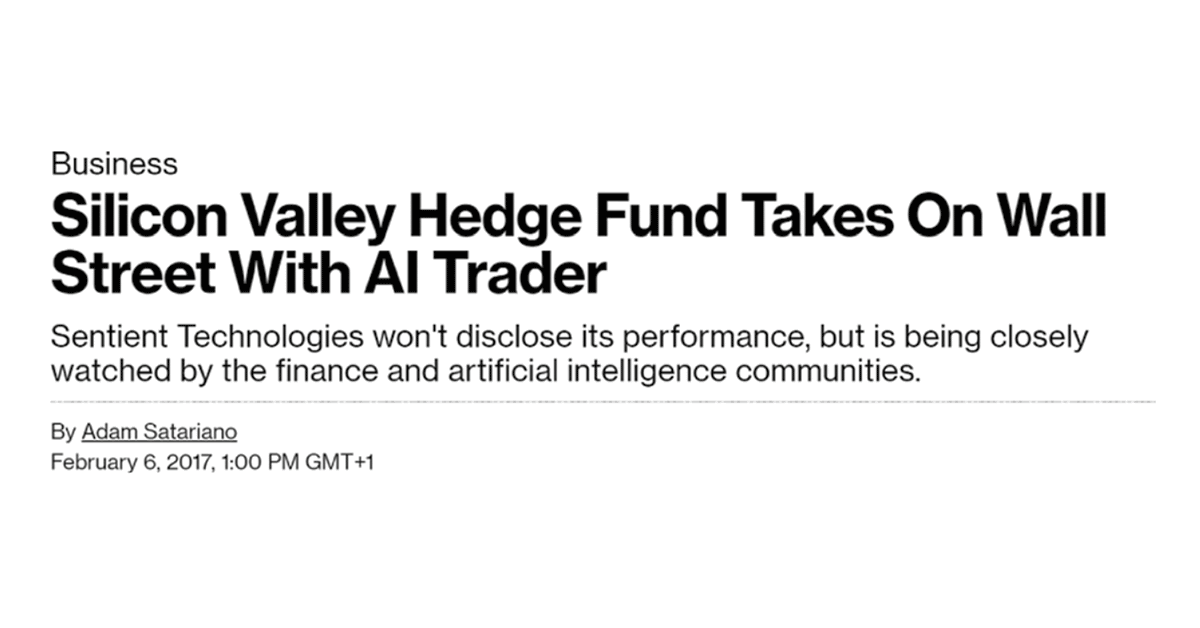
Investorbots: Too Good to Be True?
Machine learning models aren’t likely to replace human stock-market analysts any time soon, a new study concluded.
What’s new: Wojtek Buczynski at University of Cambridge and colleagues at Cambridge and Oxford Brookes University pinpointed key flaws in prior research into models that predict stock-market trends. Neither the algorithms nor the regulators who oversee the market are ready for automated trading, they said.
How it works: The authors surveyed 27 peer-reviewed studies published between 2000 and 2018 that used machine learning to forecast the market. They found patterns that rendered these approaches inadequate as guides to real-world investment.
- Prior studies often trained up to hundreds of models based on a single architecture and dataset. Then they tested the models and presented the best results. A real-world investment fund that tried the same thing wouldn’t earn the optimal return. Moreover, if it advertised its best return, likely it would run afoul of the law.
- Where real-world investors can provide a rationale for any trade, many of the proposed models were black boxes that shed little light on how they made a given decision. This lack of transparency also raises regulatory concerns, the authors said.
- The best-performing models predicted correctly whether a stock’s price would rise or fall over 95 percent of the time. That may be a high percentage, but for an investor who holds a high stake, an incorrect prediction can be a huge risk.
- Most of the studies didn’t account for trading costs, which can cut substantially into an investor’s profits.
Behind the news: Although investment funds that claim to use AI have garnered attention, so far they’ve generated mixed results.
- Sentient Investment Management, a hedge fund that used algorithms to control its trading strategies, started in 2016 and gained 4 percent the following year. It failed to make money in 2018 and promptly shut down.
- Rogers AI Global Macro ETF, an AI-driven international fund, launched in 2018 and liquidated its holdings the following year.
- EquBot’s AI Equity ETF, powered by IBM’s Watson, is “the closest we have come across to an AI fund success story to date,” the authors said. It has consistently underperformed the Standard & Poor’s 500, an index of the most valuable U.S. companies.
Why it matters: If machine learning can make predictions, why can’t it predict market activity? A couple of reasons stand out. This paper examines the misalignment between AI research and the likely challenges of real-world deployment. Moreover, even if an algorithm predicts market dynamics accurately within the short term, it will lose accuracy as its own predictions come to influence sales and purchases.
We’re thinking: Studying algorithms that make trading decisions has always been a challenge, since traders tend to keep information about successful algorithms to themselves lest competitors replicate them and dull their edge. Hedge funds that have access to non-public data (for example, specific online chats) have used machine learning with apparent success over years. But those funds haven’t published papers that describe their models!
A MESSAGE FROM DEEPLEARNING.AI
![]() |
Ready to deploy your models in the real world? Learn how with the TensorFlow: Data and Deployment Specialization. Enroll today
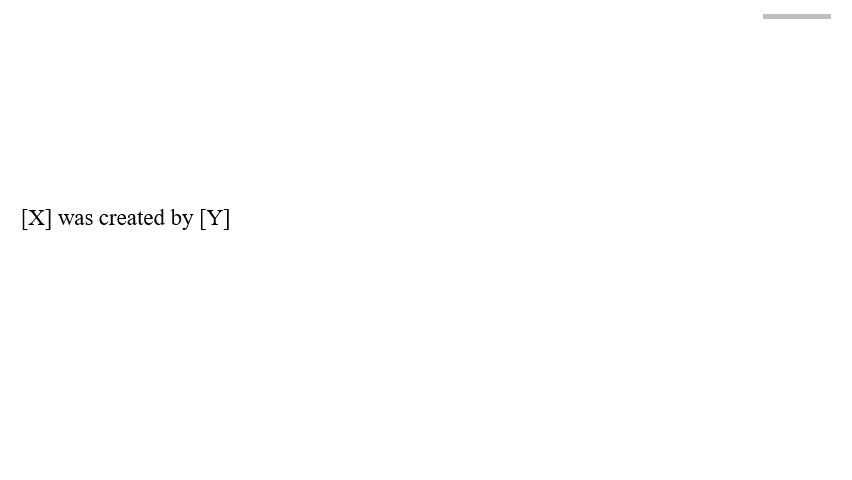
Coordinating Robot Limbs
A dog doesn’t think twice about fetching a tennis ball, but an autonomous robot typically suffers from delays between perception and action. A new machine-learning model helped a quadruped robot coordinate its sensors and actuators.
What's new: Chieko Sarah Imai and colleagues at University of California devised a reinforcement learning method, Multi-Modal Delay Randomization (MMDR), that approximates real-world latency in a simulated training environment, enabling engineers to compensate for it.
Key insight: Most robot simulations wait for the machine to take an action after a change in its surroundings. But in the real world, it takes time for a sensor to read the environment, a neural network to compute the action, and motors to execute the action — and by that time, the environment has already shifted again. Simulating the latency of sensors that track position and movement during training helps a model to learn to adjust accordingly, but that doesn’t account for lags due to reading and processing visual sensors. Simulating a separate latency for vision should address this issue.
How it works: The authors trained their system to compute optimal angles for a simulated robot's joints using the reinforcement learning algorithm proximal policy optimization. The virtual robot traversed uneven virtual ground between box-like obstacles in a PyBullet simulation.
- During training, the authors maintained a buffer of 16 frames from a virtual depth camera. They split the buffer into quarters and randomly selected a frame from each part to simulate variable latency in real-world depth perception.
- Similarly, they buffered position and movement sensor readings, for example, the angles of the robot’s joints. For fine variation over the latency, they chose two adjacent readings at random and interpolated between them.
- Selected frames of depth information went to a convolutional neural network, and position and movement sensor readings went to a vanilla neural network. The system concatenated the representations from both networks and passed them to another vanilla neural network, which generated target angles for each joint.
- The reward function encouraged the virtual robot to keep moving forward and not to fall while minimizing the virtual motors’ energy cost.
Results: The authors tested a Unitree A1 robot in the real world, comparing MMDR with alternatives they call No-Delay and Frame-Extract. No-Delay used only the four most recent frames as input. Frame-Extract was similar to MMDR but used the initial frames from each of the buffered sequences. MMDR was consistently best in terms of steps traveled through a variety of terrain. For example, in nine forest trials, the robot using MMDR moved an average of 992.5 steps versus 733.8 steps for No-Delay and 572.4 steps for Frame-Extract.
Why it matters: Robots in the wild often face different mechanical and environmental conditions than a simulation can reproduce. To build autonomous machines that work in the real world, it’s critical to account for all kinds of latency in the system.
We're thinking: Roboticists and mechanical engineers who work with physical robots have been accounting for various control latencies for decades. But much of the recent activity in reinforcement learning has involved simulated environments. We’re glad to see researchers working to bridge the sim-to-real gap and address the challenges of working with physical robots.
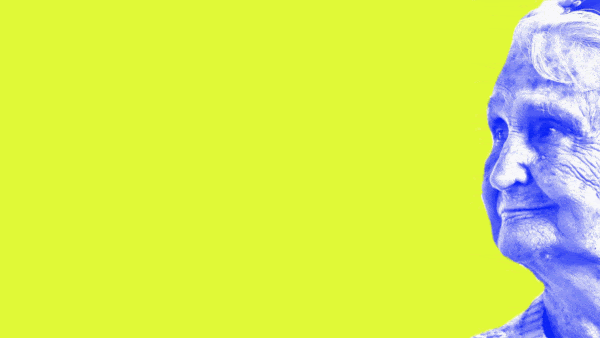
Algorithms for the Aged
The United Nations office that promotes international public health advised AI practitioners to pay closer attention to elders.
What’s new: A report by the World Health Organization (WHO) warns that elders may not receive the full benefit of AI in healthcare. It highlights sources of bias in such systems and offers recommendations for building them.
What it says: The report calls attention to four primary issues: datasets, technological literacy, diversity of development teams, and virtual care.
- The datasets used to train healthcare systems frequently underrepresent older people. They may not account for broad variations in elder health and lifestyle.
- Older people are less eager than younger people to adopt new technology, and that may affect their access to AI-assisted care. Their reticence may lead developers to view them as less relevant to the market and focus on serving younger people.
- Development teams dominated by younger people may build products that are biased against elders or don’t address their needs, particularly those of elders in socially marginalized groups.
- AI applications that automate care or monitor a patient’s health remotely may reduce contact between caregivers and patients. This may deprive elders of human contact and deepen the disconnect between young developers and older patients.
Recommendations: The authors recommend that datasets be audited for ageism, teams include a variety of ages, elders be involved in product design, elders have rights to consent and contest use of AI systems in their own care, and AI products undergo rigorous ethics reviews.
Behind the news: Relatively little research has examined age bias in AI systems. Nevertheless, elders themselves have complained about some existing systems.
- Users criticized QuietCare, a bracelet that uses AI-enhanced motion detection to recognize when a user has fallen or needs emergency medical help. They claimed that the system didn’t suit their routines and generated false alarms.
- Researchers found a dramatic gap between elders and their adult children in their acceptance of an in-home computer vision system designed to detect falls. The children were far more enthusiastic than their parents. The children also tended to underestimate their parents’ competence.
Why it matters: Learning algorithms have a well-documented history of absorbing biases from their training data with respect to ethnicity, gender, sexual orientation, and religion. It stands to reason that they would also absorb biases with respect to older people — a population that, like the very young, is at greater risk of illness and injury and generally needs greater care than the general population.
We’re thinking: Let’s do what we can to ensure that the benefits of AI are shared fairly among all people.