Dear friends,
I spent Sunday through Tuesday at the CVPR computer vision conference in Vancouver, Canada, along with over 4,000 other attendees. With the easing of the pandemic, it’s fantastic that large conferences are being held in person again!
There’s a lot of energy in computer vision right now. As I recall, the natural language processing community was buzzing about transformers a couple of years before ChatGPT revolutionized the field more publicly. At CVPR, I sensed similar excitement in the air with respect to computer vision. It feels like major breakthroughs are coming.
It is impossible to summarize hundreds of papers into a single letter, but I want to share some trends that I’m excited about:
Vision transformers: The Batch has covered vision transformers extensively, and it feels like they’re still gaining momentum. The vision transformer paper was published in 2020, and already this architecture has become a solid alternative to the convolutional neural network. There are complexities still to be worked out, however. For example, whereas turning a piece of text into a sequence of tokens is relatively straightforward, many decisions need to be made (such as splitting an image into patches, masking, and so on) to turn an image processing problem into a token prediction problem. Many researchers are exploring different alternatives.
Image generation: Algorithms for generating images have been a growing part of CVPR since the emergence of GANs and then diffusion models. This year, I saw a lot of creative work on editing images and giving users more fine-grained control over what such models generate. I also saw a lot of work on generating faces, which is not surprising, since faces interest people.
NeRF: This approach to generating a 3D scene from a set of 2D images has been taking off for a while (and also covered extensively in The Batch). Still, I was surprised at the large number of papers on NeRF. Researchers are working to scale up NeRF to larger scenes, make it run more efficiently, handle moving scenes, work with a smaller number of input images, and so on.
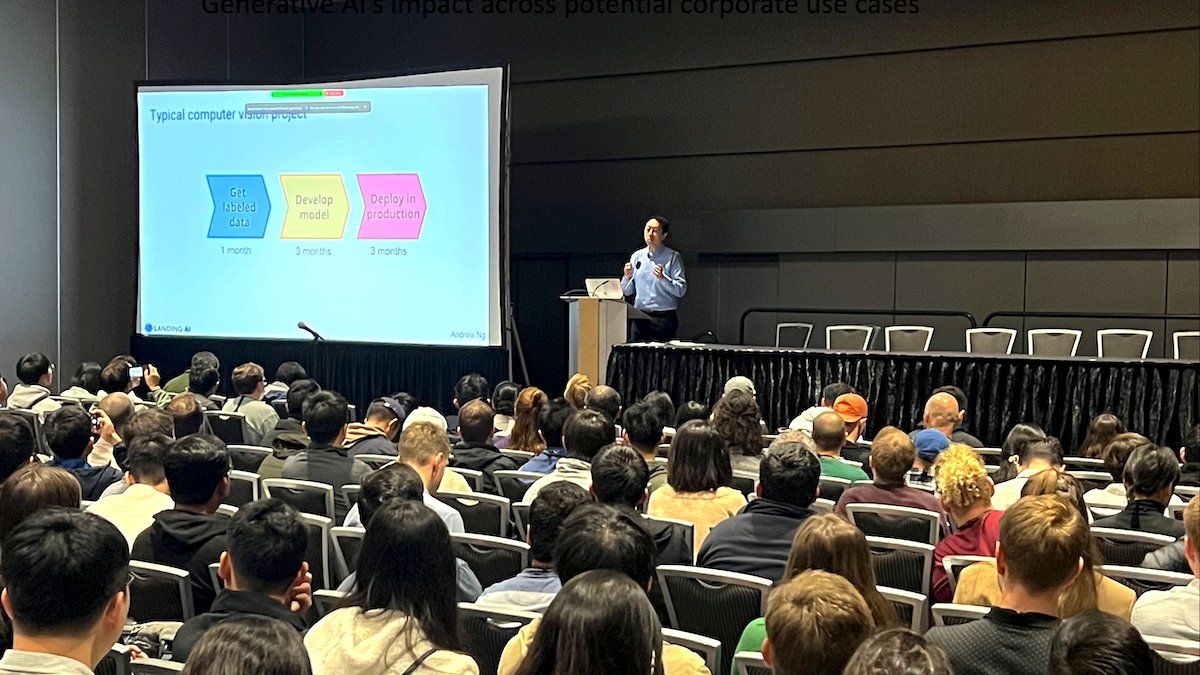
Although it was less pronounced than excitement around the topics above, I also noticed increased interest in multimodal models. Specifically, given that a transformer can convert either an image or a piece of text into a sequence of tokens, you can feed both types of tokens into the same transformer model to have it process inputs that include both images and text. Many teams are exploring architectures like this.
Lastly, even though the roadmap to self-driving cars has been longer than many people expected, there remains a lot of research in this area. I think the rise of large, pretrained transformers will help kickstart breakthroughs in self-driving.
I also spoke at the CVPR conference’s workshop on Computer Vision in the Wild about Landing AI’s work on making computer vision easy, with visual prompting as a key component. (Thank you Jianwei Yang, Jianfeng Gao, and the other organizers for inviting me!) After my presentation, speaking with many users of computer vision, it struck me that there’s still a gap between the problems studied/benchmarks used in academic research and commercial practice. For example, test sets are more important in academic research than in practical applications; I will write more about this topic in the future.
To everyone I met in person at CVPR: Thank you! Meeting so many people made this trip a real highlight for me.
Keep learning!
Andrew