Working AI: How a Former Academic Helped Launch a Company That Makes Cities Healthier
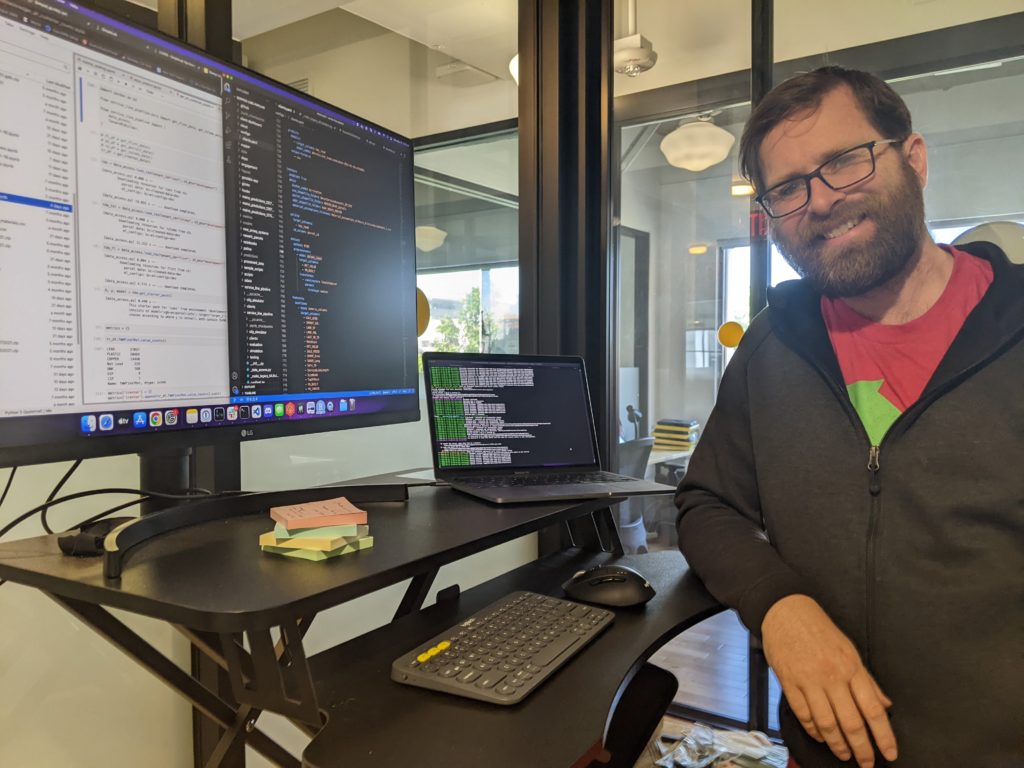
Jared Webb is the head of data science at BlueConduit, a company that uses machine learning to locate lead pipes — which can leach the highly toxic material into drinking water — in cities where old infrastructure poses a lurking hazard to residents. He helped invent its core technology as a graduate student and now leads a team of data detectives. He spoke to us about his transition from academia to business, how he navigates the challenges of working with government data, and what he looks for in new hires.
Name: Jared Webb
Title: Chief data scientist, BlueConduit
Location: Berkeley, California, United States
Education: Master’s of Science, Applied Mathematics, Brigham Young University
Hobby: Making traditional Meso-American food.
How did you first get interested in AI?
Jared Webb: Back in the mid 2000s, I was an aspiring mathematician, but didn’t really know what kind of math I wanted to do. I was initially interested in a PhD in applied mathematics, focused around topics like simulation and portability. The most exciting applications were focused on data and AI. I saw these beautiful mathematical results getting turned into products that represent the real world. Even better, the results could help make the world a better place. Eventually the applications became more interesting than the math, so I abandoned the PhD in favor of working to solve these complex social and infrastructure problems.
How did you get involved with BlueConduit?
Around 2014, when the Flint Water Crisis happened, I was part of a research group at the University of Michigan brought on to advise the city because their lead pipes were releasing toxins into residential drinking water. But they also had an information crisis, because they didn’t know which homes were served by lead pipes. I was one of several researchers brought on to help sort things out with data science and machine learning.
We trained a machine learning system that estimates which homes received their water from lead service lines using city records, information on pipe records, and thousands of water samples. This allowed city officials to efficiently allocate their resources, only digging where pipes need to be replaced.
We started going to city council meetings and realized that it wasn’t just an academic problem. The city council members would put our results on the board, and afterward people would approach us. It became clear that these weren’t just lines in a spreadsheet, these were human beings. We wanted to help anybody we could.
Then the Atlantic wrote a story about us and other academics started paying attention to the work we had published. As a result, officials from other cities started contacting the University of Michigan saying they had the same problem as Flint. They wanted our help.
While we were able to map Flint’s lead pipe problem for free, that just wasn’t scalable. If we wanted to bring this technology to other cities then we would have to form a company. So in 2019 we created BlueConduit.
What is your day-to-day work like?
We have successfully deployed our technology in several places now, and that means we’ve been able to grow. I manage a team of awesome, talented data scientists. They’re on the front lines, building and deploying models. My day-to-day is helping them when they are stuck, acting like a tiebreaker, mentoring them, and helping everyone learn more about this problem of lead water pipes.
One of my roles is also to make sure our claims are precise and not exaggerated. I see this issue in a lot of marketing, where people claim AI can do everything. I work with leadership and clients to make sure nobody is dazzled by words like “AI” and “machine learning”. I make sure everybody has a very grounded understanding of what we are doing.
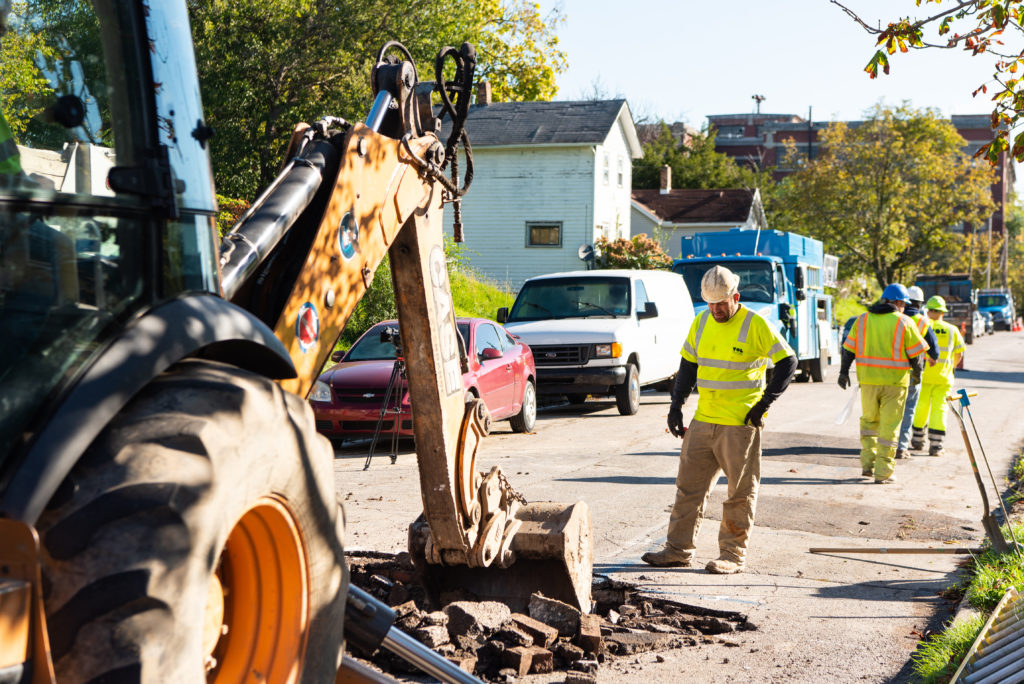
What were you least prepared for about making the transition from academia to business?
In the academic workflow, you explore questions more freely and chase all sorts of interesting ideas. In contrast, in the business workflow, you set goals and you have to deliver certain results. If you find something interesting along the way, you might have to abandon it if the people paying you don’t also find it interesting. Working within these constraints and being customer-focused introduces new, intellectually-satisfying challenges.
What do you look for when you’re hiring data scientists?
I primarily look for people who understand the constraints of data. Especially government data, which is messy. A good way to get experience working with messy data is to take internships and capstone projects with government agencies and nonprofits. That kind of practical experience is, to me, more valuable than projects where you, for instance, build a neural network that can see an oncoming car using terabytes and terabytes of cleaned image data. Most government organizations have a lot of data, but it is not clean and it is usually never for the exact problem you need to solve.
Any red flags that alert you when to not hire somebody as a data scientist?
I’ve come across resumes claiming that, during a semester-long internship, the applicant implemented a machine learning model that saved their company millions of dollars. I don’t believe it. Almost always those claims are being made against unrealistic baselines, like random guessing, that don’t reflect the reality of what an expert in the field would do without a machine learning model.
You mentioned that government data is messy. Do you encounter any other challenges particular to using government data?
There are interesting bureaucratic constraints. You might hear about a dataset that exists. But then you might need to talk to a certain person in a certain department to get access to that data, and finding that person sometimes takes awhile.
Other constraints include communicating what should be done with the data. I can’t just email people a list of probabilities. I’m often helping our clients interpret and understand the data both within the context of their problem and the constraints of their budget.
Several research groups have flagged brain drain as a problem in AI, due to the number of academic researchers being lured into private-sector work. What is your view on this phenomenon?
I see the crossover between academia and the private sector as a positive. I’m not currently, nor have I ever been, faculty, but it can be really good for universities to have their research spun out into the real world. Especially if it has some kind of immediate impact. In the U.S, public universities were often founded as land-grant schools with missions of helping farmers develop more effective agricultural practices. If universities encourage their faculty to do things like this in the broader world it can improve the school’s social footprint.
How do you stay on top of new data science research?
I can’t emphasize enough how important it is to understand foundational statistics. This helps you understand when you’re experiencing real success. How you measure accuracy is so important, and you don’t want to lose that by being obsessed with building the fanciest generative adversarial network. If you understand your fundamentals, you’re less likely to mess up.
What new challenges would you like to see AI applied to?
The real question for me is: Where should we start gathering data? Basic machine learning techniques could help address a lot of social problems if we had the right data. How do we make sure that we can do that effectively, while balancing ethical and privacy concerns? At the same time, people don’t like feeling as though they are being handed over to a machine. There is a lot of work ahead of us to strike the right balance.
Connect with Jared Webb on LinkedIn!
Want to learn how to use AI to make the world a better place?