How a New Mother Learned AI During Her Newborn Baby’s Naps
Name: Apala Guha
Title: Senior machine learning compiler engineer at Lightmatter
Location: Boston, MA
Education: Bachelor of Science, Computer Science from Jadavpur University; Master of Science, Computer Engineering from the University of Virginia; PhD, Computer Engineering from the University of Virginia
Favorite ML area: Performance Optimization at AI inference
Apala Guha is a senior machine learning compiler engineer at Lightmatter, a Boston-area startup. Before that, she was a computer scientist who had always been interested in deep learning. When she quit her previous job at the beginning of 2020 to have a baby, she took advantage of the “time off” to take the Specialization. She talked to us about how she found time to keep learning while caring for an infant, how her knowledge of AI helps with designing computer chips, and why the Deep Learning Specialization helped her feel emotionally connected to her profession for the first time.
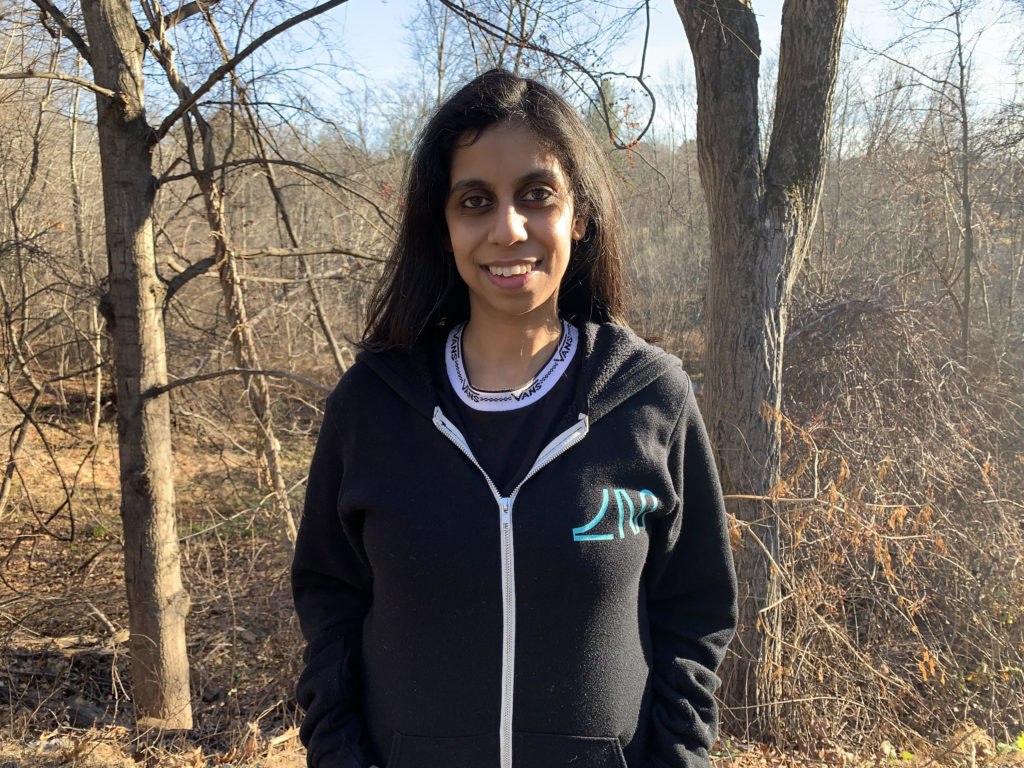
What were you doing in your career before you started working in AI?
I have a Ph.D. in computer science, and have worked at Intel and as a researcher for several universities. At first, my work had nothing to do with artificial intelligence, but over time AI came to the forefront, and eventually, our focus shifted to building systems specifically for AI. I wanted to take the Specialization for a while but didn’t have time until I stayed home after my baby right after the pandemic started.
How were you able to take the course while also caring for a newborn?
I had quit my job right before having the baby, so taking care of it was my new full-time job. But I was a bit concerned about being out of touch with work during that time and thought about how to use my free time productively. Luckily, the content in the Specialization was delivered in small bites, which is all that I had. Yes, caring for newborn babies is a full-time job, but they also sleep a lot. I did not have control of when the baby would sleep or for how long, but I developed a mindset that I could work around this uncertain schedule. The baby would nap, I would make a little bit of progress, and I would keep doing that day after day.
Given your prior experience working in computer science, why did you feel it was necessary to take the Specialization?
I have spent most of my career working from the system’s perspective. I also taught data science, but mainly focusing on data mining. I had never worked on anything purely machine learning, and I felt that this was the right time to do so. I was building systems for machine learning and felt that I could even use machine learning to help build the system. It seemed to be the highest priority thing I could learn at that time.
How challenging was it for you to find work after your maternity leave was over?
I didn’t have any problems. I interviewed with three companies and got two job offers. This was my first job after having the baby and taking a long break, but my interviews went very smoothly. I attribute a lot of that to the sound fundamentals in deep learning that I could obtain in such a short time.
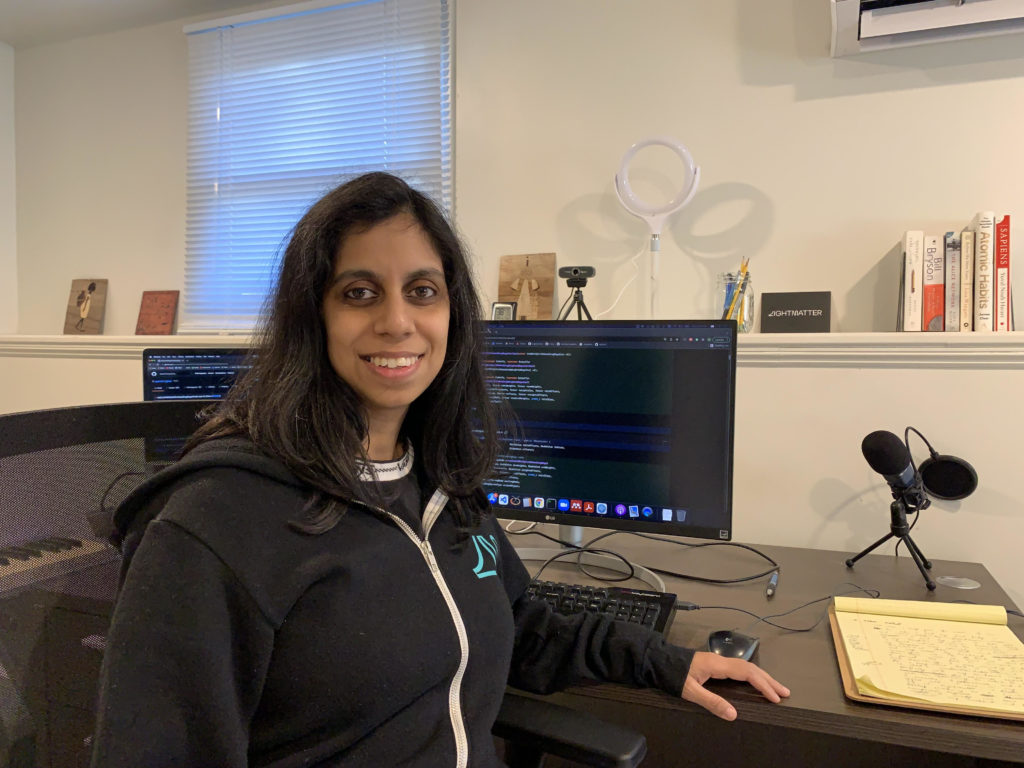
Can you tell us a little bit more about your current role?
I work in a Boston-based startup called LightMatter, building AI inference accelerators using optical computers, which use light as a signal instead of electricity. That’s what we’re doing at the hardware level. At the application level, we are targeting AI, primarily deep learning. It has been around for three years, and I’ve been working here for six months. As for my specific role, I am on the compiler team. My job is to take, say, a deep learning network written in PyTorch or TensorFlow and port it over to our hardware. So I need to have a pretty good understanding of deep learning fundamentals, and the Specialization helped me get this job.
Were there any aspects of the Specialization that you found particularly impactful?
From my perspective, I want to be a user of AI but will probably not invent some new type of architecture. It was great at serving me precisely what I needed and leaving out the things I didn’t. For example, the material explains things without going too deeply into mathematics, which would have been hard for me to absorb in such short bites of time. I understand at high-level things like why Resnet has skipped connections or how YOLO makes single shot detections without being bogged down by the nitty-gritty details of every little thing. Andrew is a great teacher, and he did a fantastic job explaining concepts by relating them to things we already understand.
Are there ways that the Specialization helps you in your day-to-day work?
It definitely helps to fast-track my projects. You don’t necessarily need an understanding of deep learning to build these computer systems, but it would take a lot longer. I either already know about the networks I am working on in my projects, or I can go and review lectures to familiarize myself. I don’t need to speed days or weeks understanding how a network does something. As a startup, we don’t have a lot of people, so saving time is very important.
How satisfied are you with your career now compared to before taking the Specialization?
Before taking the course, I was a computer scientist and have always worked with computer systems. But I struggled to find an application that I could connect with. Since returning to work and designing computers specifically for AI, I have felt more passionate about what I do because I feel connected to what is going on in society. For example, if face recognition is in the news, I feel connected to the discussion because the system I’m building does face recognition. I feel very passionate about these issues because they relate directly to me.
Ready to #BeADeepLearner like Apala? Enroll in the Deep Learning Specialization
You can find Apala Guha on LinkedIn.