Answers from the instructor: AI for Good’s Robert Monarch
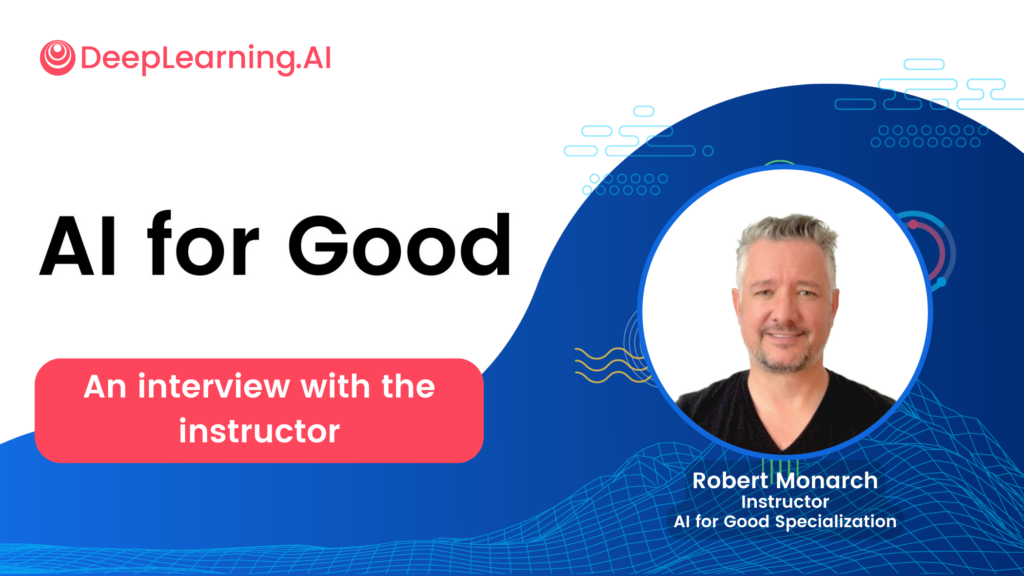
The world is filled with complex problems: Pandemics, natural disasters, and armed conflict, to name a few. Professionals and volunteers working to address these challenges are increasingly finding uses for AI. Our new specialization, AI for Good, aims to expand the pool of people with the motivation and technical expertise to combine human and machine intelligence for positive outcomes.
AI for Good’s instructor, Robert Monarch, has spent much of his life pursuing two careers in parallel: machine learning and disaster response. On the machine learning side, he has worked for tech giants like Apple and Amazon, as well as co-founded his own startups. On the humanitarian side, he has worked in post-conflict development for the UN, helped create an archive of endangered languages, coordinated emergency response in the aftermath of the 2010 Haiti earthquake, and designed a system for monitoring for disease outbreaks that was used for COVID-19. Robert earned his PhD from Stanford University, where he focused on adapting natural language processing for healthcare and disaster response. He is the author of Human-in-the-Loop Machine Learning, from Manning Publications.
He spoke with us about his career, where he’s seeing AI applied to social and environmental challenges, and how others can help join the growing AI for Good movement.
What is your background?
I’m currently a machine learning professional and have been working in Silicon Valley for about 15 years. I’ve been with big companies, like Amazon Web Services and Apple, and startups focused on data labeling and machine learning.
Immediately before moving to Silicon Valley I worked for the UN High Commission for Refugees in West Africa and before that I worked with indigenous communities trying to document and preserve their languages. Since moving to Silicon Valley I’ve continued to help with responses to earthquakes, floods, tsunamis, wildfires, and several pandemics, including Covid-19.
How did you first get involved in machine learning?
When I was a college undergraduate I started taking computer science courses after hearing about this thing called the Internet. At that time, I thought AI only existed in sci-fi novels, but my college had a course on it, which I took and enjoyed. Around two years later, while still an undergrad, I published a paper at a leading data mining conference. I enjoyed that experience but didn’t expect to make a career out of AI. At the time it seemed like the only AI jobs were doing government surveillance, which I wasn’t interested in.
What catalyzed you to finally pursue a career in AI?
It wasn’t until after completing my undergraduate degree and doing humanitarian work that I encountered real world applications for AI that interested me.
When working for the UN in Liberia, my co-workers and I heard that there were some refugees in a neighboring valley.
We were unable to find out exactly how many people were there and what help they needed, which was especially frustrating because many of those people would have had cell phones that were probably connecting to the same phone towers as mine. Even if we could have contacted them, they could have spoken one of a dozen languages that we did not share, and they would have limited ways to help themselves because machine learning that we took for granted 20 years ago, like search engines and machine translation, didn’t exist for those languages.
When I then moved to the US to pursue my PhD at Stanford University, those experiences and continued disaster response efforts became the focus of my research: How do we apply natural language processing to text messages in healthcare and disaster response situations?
How have you gone on to put this research into practice?
I think I made some of my biggest impacts developing tools that allow communities to help themselves. For example, when I led the effort for AWS’s first natural language processing product, Amazon Comprehend, I fought hard to make it language independent from the start. This has made it a lot easier for non-English communities to bootstrap off this technology and build out their own capabilities, and helped ensure that we minimized the assumptions that might have been hard-coded into an English-only system at launch. That may seem pretty abstract, but I think providing that sort of baseline technology is one of the most important things we can do in disaster response.
Throughout my disaster response work, I’ve seen how much people struggled with access to information. Especially people who speak languages with just 10,000 or even 100,000 other speakers. We take this for granted in the English-speaking world, but things like search engines and speech recognition still don’t exist for a majority of the world’s languages.
What can people expect to learn from the AI for Good Specialization?
I think that working with at-risk communities has helped me become a better machine learning leader in general, because many of the same risks and potential biases that exist in disaster response contexts exist in many other application areas, but are not always so obvious. The opposite is also true — my experience building products that utilize AI in industry has helped me understand the importance of using reliable technology in disaster response contexts.
So, while the course is focused on humanitarian and environmental projects, people who take this specialization can expect to learn how to ship any product that utilizes AI. They will learn how to evaluate which type of AI to use for their goals, and whether using AI is appropriate. We focus on specific use cases, for example addressing things like climate change, disaster response, and healthcare. People will also learn about humanitarian use-cases for AI that they might not be aware of. The coursework builds as much on my time in Silicon Valley as it does my work in disaster response.
Finally, the specialization provides a strong warning against cases which might not have a positive impact. AI has the potential to provide immediate positive effects on people, but by that same token mistakes can very easily cause harm to people or the environment. We teach people how to avoid these cases, for instance by showing them how to be careful with data and alerting them to sensitive use-cases. This might be the only machine learning course that argues that you should not try to build most of the ideas that you come up with, despite your good intentions.
What are some general bits of advice about deploying AI in humanitarian situations?
In my background as a disaster responder, I’ve found that AI can have its biggest impact when it is improving the pace and quality of human actions. For example, after a hurricane you can use computer vision to identify missing bridges and other breakdowns in your logistics network. You can also use it on sensor data to identify structural damage that might not be visible to the human eye. It can help firefighters identify wildfires earlier. It can help get information out to people who speak less-common languages. In all these use cases, it is still a person who is the decision maker and the AI is assisting their work.
In humanitarian work, just as in other areas, subject matter expertise is really important. If you are already volunteering, for example, for a health clinic in a non-AI role, you should use your experience to think about ways you can apply AI to help the clinic be more effective. If you speak a less-widely-spoken language, you should work with that language community to find applications.
When working in disaster response, I always want to be sure that whatever AI model I am using is reliable. I want to know that it has been used in a large number of other contexts. You want to send tried and true software to support humanitarian missions for the same reason you want to send a Land Cruiser, and not a prototype SUV, into a disaster zone.
Most importantly, you don’t want AI to make things worse. You want to evaluate your AI system ahead of time to make sure it won’t cause harm or damage that wouldn’t have existed otherwise.
What prerequisites does somebody need to have before taking the specialization?
You need an understanding of the basic principles of machine learning, but you don’t need to be an engineer. This specialization looks at AI from a product development standpoint; You aren’t going to be building models from scratch, you’re evaluating how AI can be helpful in a given context and using a framework to deploy your system.
What can people from a humanitarian or environmental background expect to get out of this specialization?
If someone comes from a humanitarian background they will already have a good intuition about what tasks are good targets for automation. The specialization will help them ground those expectations with a clear understanding of what AI can and cannot do to help.
How do you keep learning?
On the machine learning side, I think it’s actually easier to keep up with things today than it was 10 years ago. Back then, we had dozens of different sets of algorithms each specialized for different tasks. Today, there is a much smaller handful of neural network techniques that are seeing practical use, so a broader range of machine learning researchers and practitioners can work on developing the same technologies.
Keeping up with the humanitarian applications is more difficult. I am regularly seeing use cases for AI that I had never considered before. For example, I grew up in Australia where we have a lot of wildfires. Early detection is crucial if you want to contain their spread, but you can’t realistically have humans constantly monitoring huge swaths of forest for signs of fire. I had never thought there might be a way of automating this process. In the specialization, however, we look at how people are using AI to identify signs of smoke from sensor data. The system then sends an alert to human reviewers, who can quickly mobilize a response. This was brand new to me, along with several of the other applications we’ve featured in the specialization. Having all these applications in one place is one of the reasons why I think AI for Good is going to be such a great resource.
Join our new specialization: AI for Good